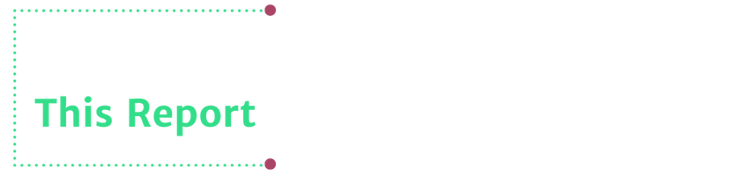
A Challenging Question
ROI sounds simple: will we get more out of this business investment than it costs?
The reality is anything but. Calculations of return on investment (ROI) usually focus on gains in productivity and efficiency – will we save money; can we raise output for the same amount of input; will revenue increase? However, making changes in complex organizations has implications that are not obvious.
Business models may need to be revised to take advantage of what an investment has made possible. New product opportunities or better ways to compete can emerge.
These effects can be hard to envisage and even harder to calculate, but it would be a fatal an error to dismiss them as secondary. Not only can they fundamentally alter ROI assessments, but failing to appreciate their impact can have lasting repercussions. The ROI of business investments can change an industry’s winners and losers, or even fundamentally alter an industry itself.
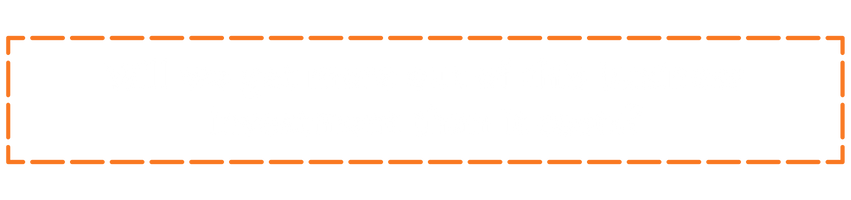
The ROI of Data in the Mortgage Industry
Data is an important driver of business costs and opportunities in many industries, not least financial services and especially mortgage lending. The mortgage industry has accepted high data costs as a fact of its business model and the means of minimizing risk in both origination and secondary markets.
Data are so pervasive and indispensable in lending that calculating the ROI of data that are the source of lending intelligence is an exceptionally difficult challenge. However, the potential impact of new data processing technologies, including automation and artificial intelligence (AI), has presented mortgage industry leaders with an ROI question they cannot avoid.
This paper considers the ROI of data in business, first generally and then in the context of the mortgage industry. It considers the role, impact and value of trusted data in contemporary business, before exploring three areas of ROI that may have a significant impact in the mortgage industry. It has been researched and written by some of the most seasoned analysts in the fields of business technology, ROI and the value of disruptive technologies including AI.
We hope that the questions and conclusions in this paper will be helpful as you evaluate the need, effects and timing of investments that will change how your mortgage business handles data.
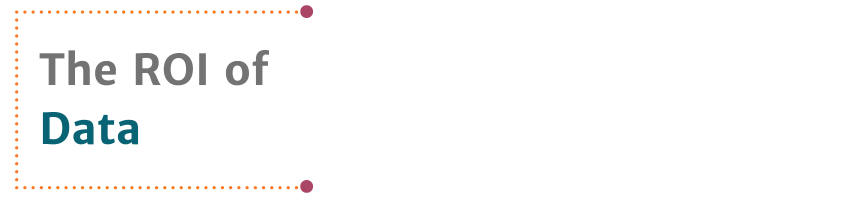
In our work with financial services organizations, we find that clean, reliable, trusted data is one of the most effective investments to deliver both immediate and ongoing ROI, including margin improvement, competitive advantage, and business growth. This paper examines how discovering, nurturing, maintaining, and exploiting clean, trusted data throughout the business enables this ROI.
Though it may seem outdated in our digitally-driven 21st century, physical documents remain a core source of essential data in many industries – the mortgage industry among them. Almost any business process that relies on physical documents or, more typically, images of physical documents (e.g., scans, photos, faxes) must handle diverse streams of unstructured data alongside structured data available from an increasing array of digital sources.
In mortgage lending, unstructured and structured data types need to be combined and coordinated to serve multiple work streams. They must be managed in accordance with exacting and frequently changing regulations, and their availability and reliability must keep pace with changing business conditions. The highest cost and source of risk for most such firms come from errors in workflows that rely on often dirty data.
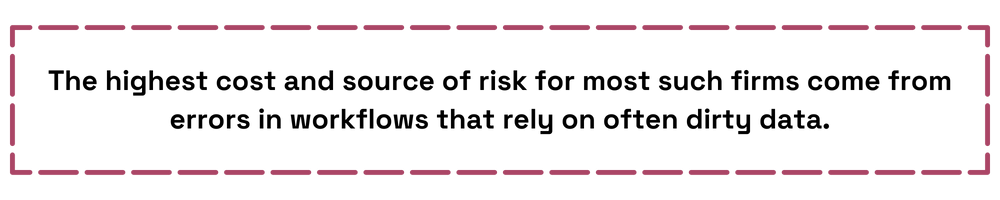
Characterizing Data
To err is human. When humans enter unstructured data into systems and processes, they can miss errors and sometimes add to them. Cassie Kozyrkov, Chief Decision Scientist at Google, has written about what she calls the Philadelphia problem that occurs when people living in the City of Brotherly Love enter their city’s name in a text field on a form but, well, they make mistakes! Here’s the list of spellings she uses to illustrate dirty data:
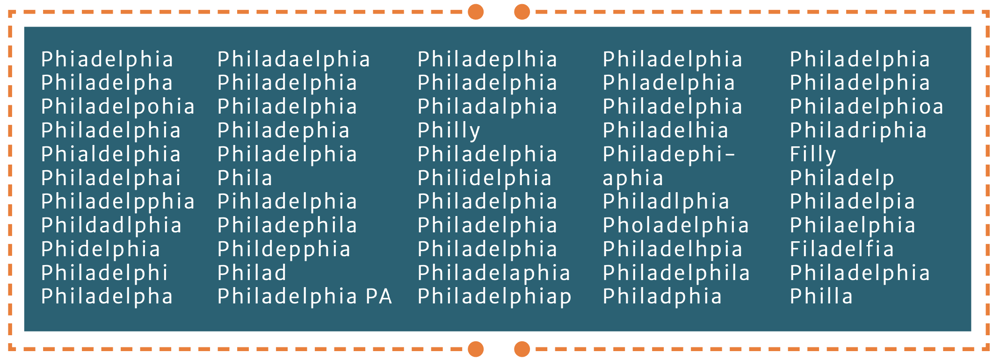
Dirty data – structured and unstructured – can be found in native and imaged documents, email messages, written survey responses, transcripts of call center interactions, or even social media posts. Dirty data are incomplete, incorrect, invalid, unreadable, irrelevant, duplicated, or improperly formatted – and usually, combinations thereof.
Dirty data are bad for business, leading to increased costs and reduced productivity, but also introducing the potential to damage reputations, harm relationships, and ultimately limit business opportunities. In short, lousy data engenders distrust in the business. And as more business processes share more data, errors, costs, complexity, and distrust are compounded.
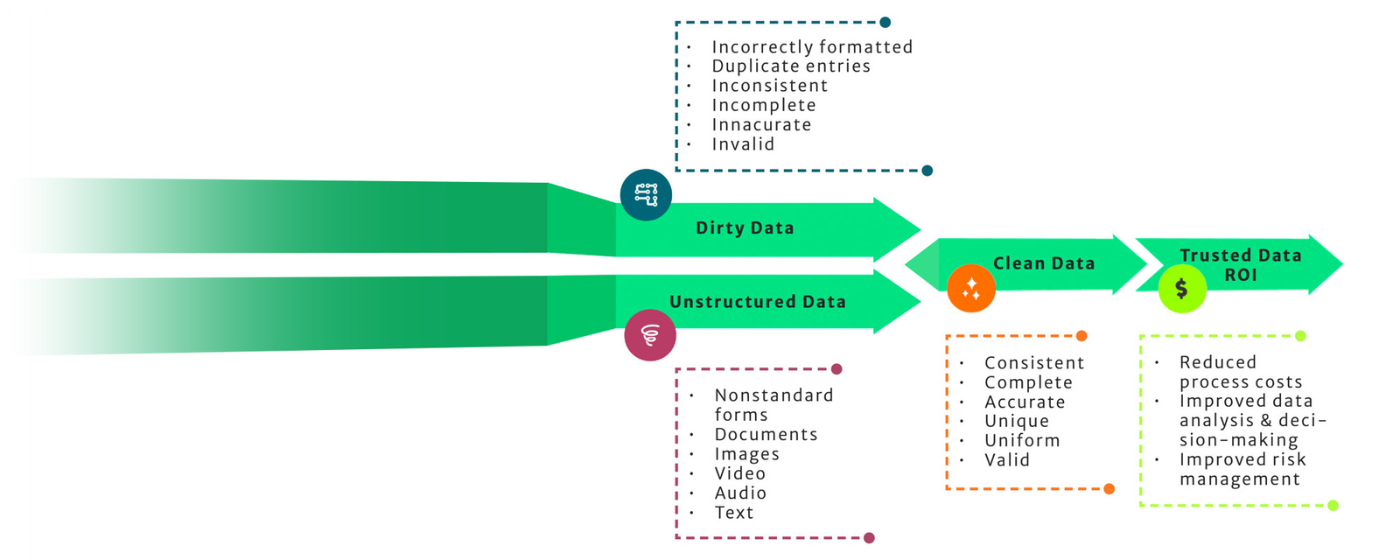
It’s a fool’s errand to assume all it takes to “solve the problem” of laborious business processes is to invest more in automation technology. Most all business processes rely on clean and readily available data; what can deliver the greatest ROI immediately and continuously? More technology is not (really) the answer. Better data is the answer, and technology can enable better data.
Data is one of the most critical business differentiators. High-quality data – rich, proprietary, accurate, and available – provides the building blocks to drive more efficient, lower-cost processes that business technology can ultimately utilize.
High quality data does not necessarily mean higher costs. Cost of quality (COQ) is a methodology used to define and measure the resources used to maintain quality and prevent product problems, as opposed to the costs resulting from product failures (whether internal or external). COQ is sum of two factors: the cost of good quality and the cost of poor quality. This is more complex that it appears: the cost of improving quality may ultimately be lower than the status quo and improved quality will reduce product failures and their associated costs.
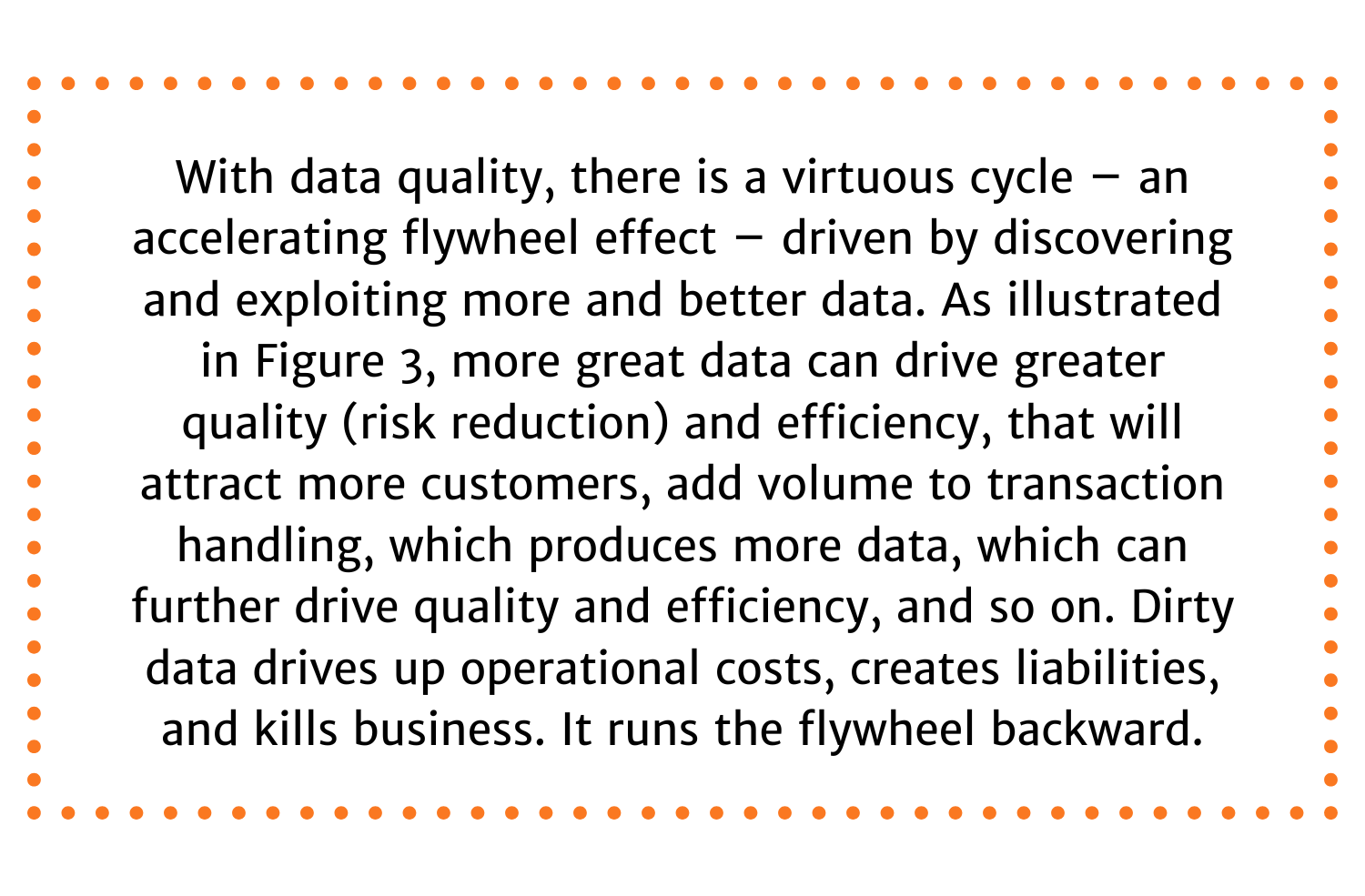
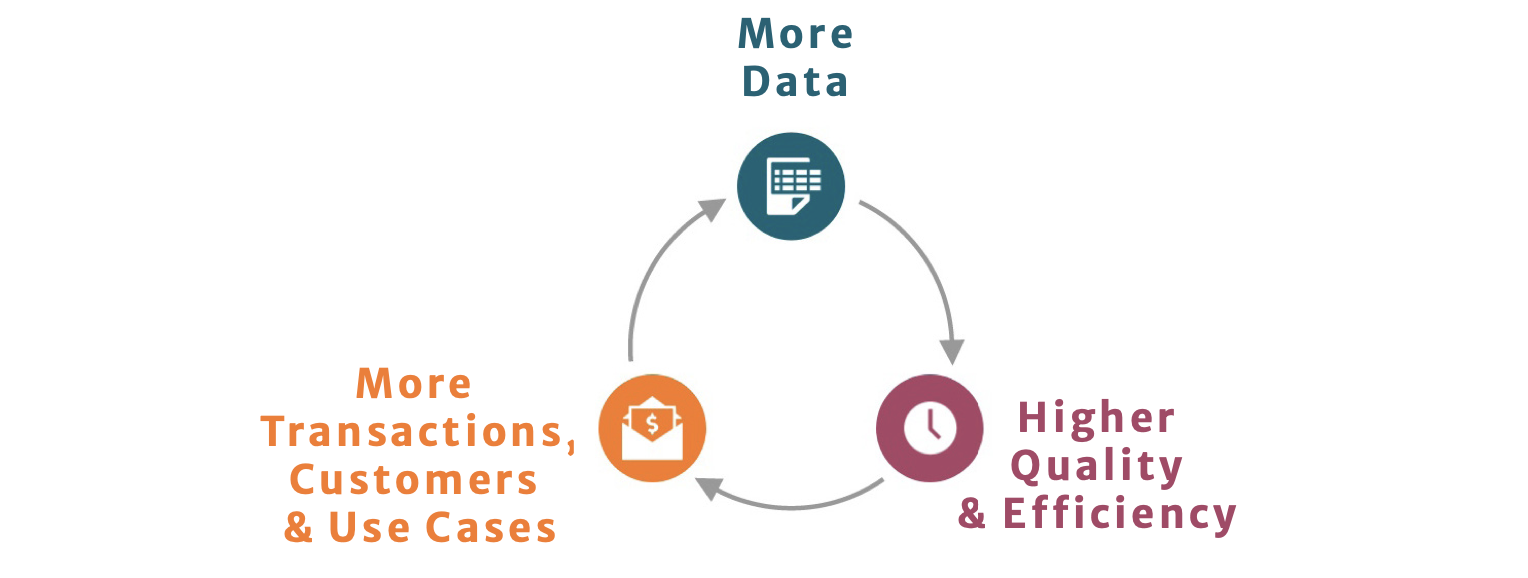
How Clean Data Create Trust, Value, & ROI
If data is to become a critical business differentiator and an enabler of continuously improving ROI, it must be reliable. Reliable data, at every stage of every process, enables firms not only to reduce operational costs and risk but also to sustain and leverage these improvements over years – and to grow more business with more profit in volatile markets.
To make data reliable – to create trust in the data – it must be cleaned. Clean data means data that are correct and consistent everywhere in the business. Data are made clean by removing or repairing the data problems listed in Figure 2. The earlier in the business process that data are made clean, the cheaper the process becomes, and the less risk the process generates.
The ROI of clean data begins immediately, and recurs and expands. For example, an upfront improvement in data cleanliness from 80 percent1 to 99 percent complete and accurate2 (or higher with the best solutions) can save the business much more than the 19 percent improvement. The clean data itself becomes a multiplier applied to every process using that data.
As that spinning flywheel of data as an asset extends more deeply into the business and trust in data grows, staff become more efficient, confident, and able to accomplish more. Many of our CFO clients report staff efficiency and productivity improvements throughout their Finance organizations as their use of clean data reduces the need for staff. involvement per process step. This frees hours of skilled labor for tasks that add value, such as sales and customer support. It also enables (and may pay for) retraining/reskilling to develop even more value from staff.
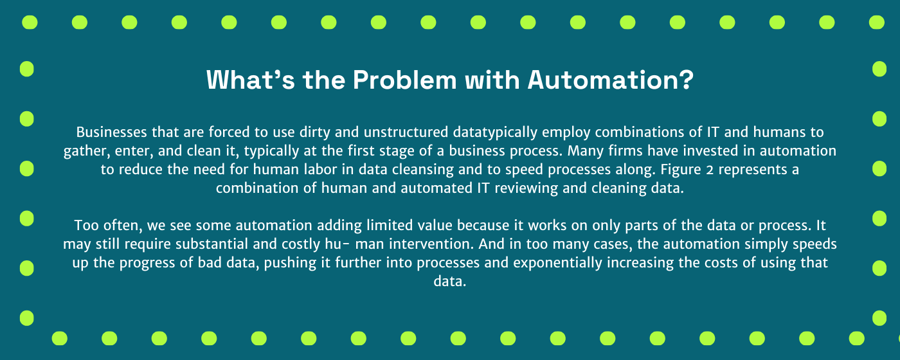
Improving Trust in Data via Self-Improving Automation
Automation should, by definition, reduce the costs of human intervention in many business processes. But we minimize or even negate ROI when we automate things that don’t already work correctly.
What happens when we effectively automate the process to correct itself? Our client work indicates an increasing scope of examples where self-improving process automation reduces the need for staff intervention and enables continuous quality improvement while increasing trust in the data itself.
Effective automation enables increasingly accurate – trusted – data at every step in as many processes as possible, from as many sources and data types as needed. Automate this intelligently, and we can develop ever-more-valuable data automatically with little to no added human cost
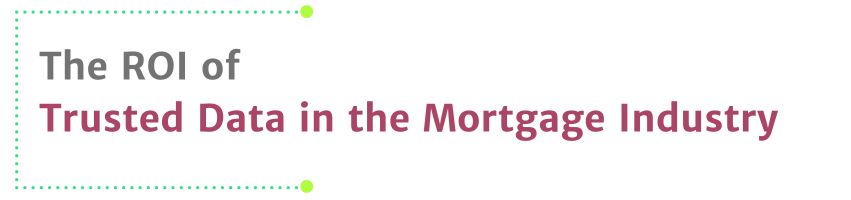
Part 1: Business Elasticity
The mortgage industry is inherently cyclical, with volumes in home buying and associated mortgage lending following well-established annual patterns. When we look at loan originations over time, as in figure 3, the quarter-to-quarter variability in volume indicates a relentless challenge for mortgage professionals to build, operate, measure, and adjust the processes to run the business at sustainable margins.
Each peak represents a burden of work for lending professionals, each valley, a lack of operational efficiency. Traversing the two bring more problems: delays for borrowers, increased risk as operations teams struggle to maintain throughput, or changes to staffing or outsourcing levels in response to rising and falling demand. Adding or removing capacity creates the conditions for future pain as changes will inevitably need to be reversed as the cycle swings back.
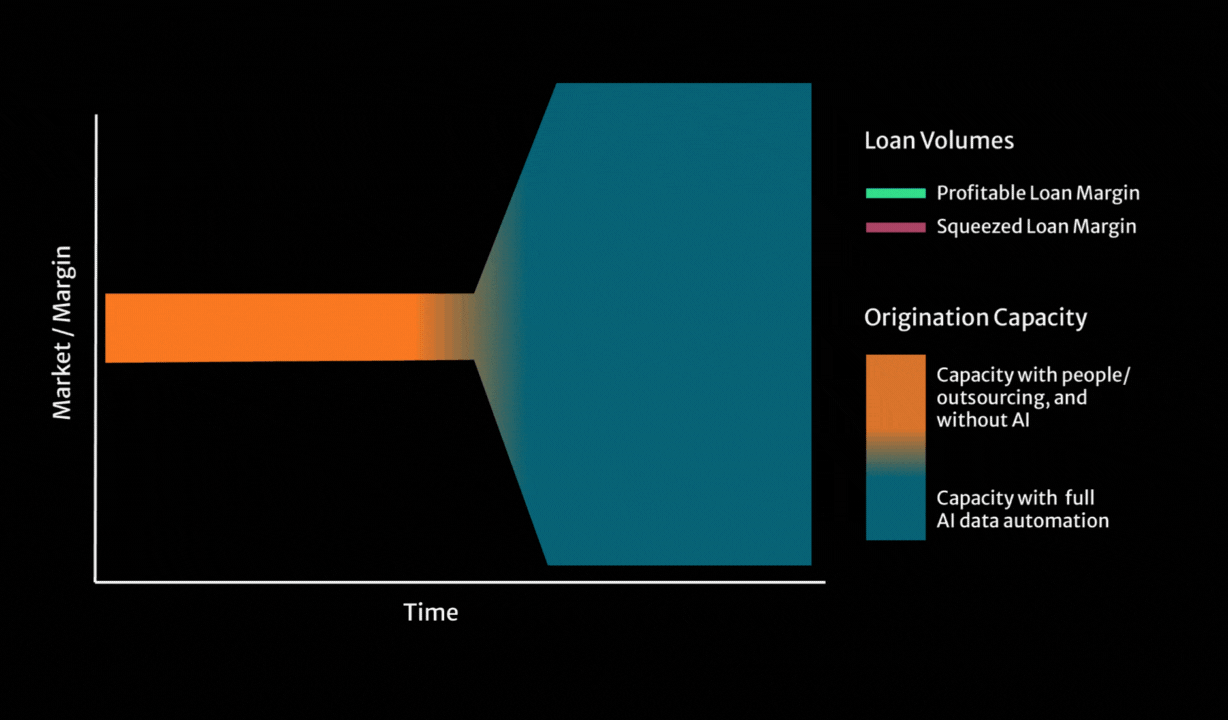
Confusing the picture are multiple direct and indirect factors: economic growth, inflation, interest rates, availability of staffing, rates of pay, new regulations, social trends, and more. These may significantly compound or moderate the effect on volumes, yet they are difficult to calculate or even know. The complexity challenges mortgage industry executives to identify what issues to focus on to manage sustainable, resilient, scalable, and efficient operations.
Mortgage lenders have long sought to improve elasticity in their business model, but productivity improvements are masked by the investment necessary to facilitate continual adaption in the face of unpredictable variability. The answer is to uncouple the relationship between volumes and staff numbers, an outcome that can only be achieved by the intelligent automation of clean data.
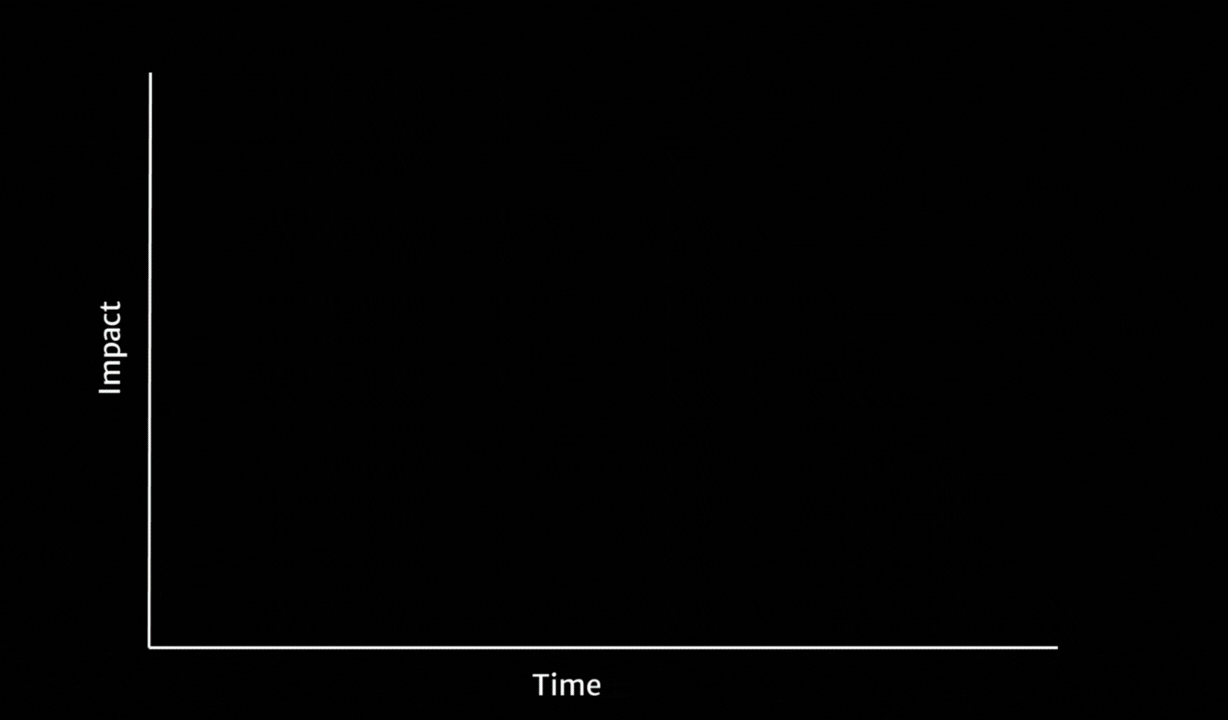
Part 2: Cost to Correct
“The sooner, the better” is a motto to live by when facing up to mistakes. It applies equally well to fixing data errors in business processes. Figure 4 illustrates how the relative cost of using bad data increases, almost exponentially, as it gets deeper into business processes. Put another way, the further data progress through workstreams, the greater its value. Conversely, the cost impact of bad data on that workstream also increases at least at the same rate.
Consider an incorrectly transcribed dollar value caused by a poorly scanned bank statement at the beginning of a loan application process.
Caught early, the cost of correcting the error is a small amount of time, and the corrected data can be used reliably throughout this process and others. Caught late, that same data error may invalidate everything from the applicant’s qualification through the underwriting – or worse – undermining the value and credibility of the loan portfolio.
Simply multiplying the time spent at each step of the process by the hourly wages of all the people involved can provide a rough estimate of the initial cost of that bad data. If the entire process needs to be restarted, those costs get incurred again. And that doesn’t account for business losses that likely occurred, such as the loss of that time to perform other work, the potential loss of the applicant’s business, or – if such errors recur enough – the potential loss of current or future business from other applicants and partners.
Intelligent automation using clean data significantly reduces the time and financial costs of errors by eliminating the need for human labor to transcribe data, and identify and correct errors. Applying automated clean data processes at multiple stages throughout the loan origination process also helps to prevent data errors from passing initial underwriting and seeping into the loan portfolio.
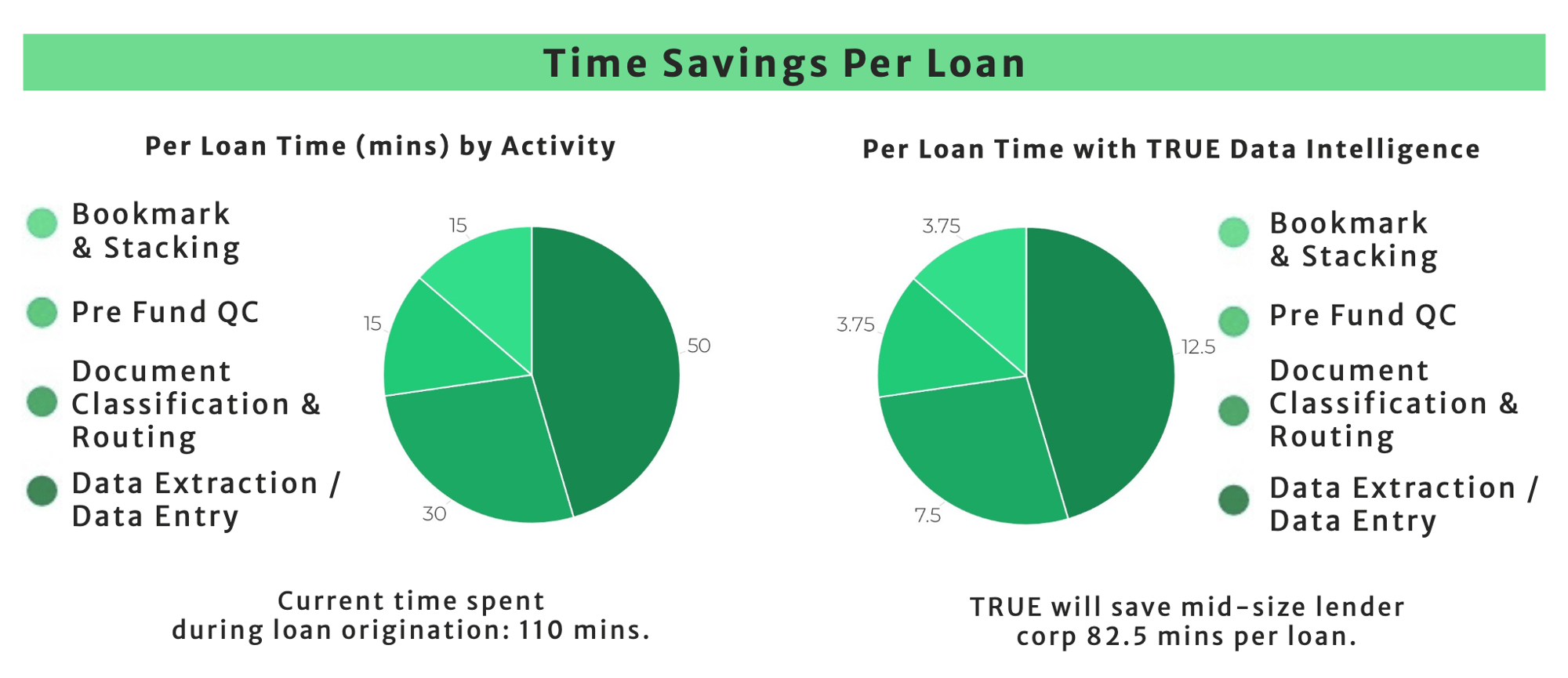
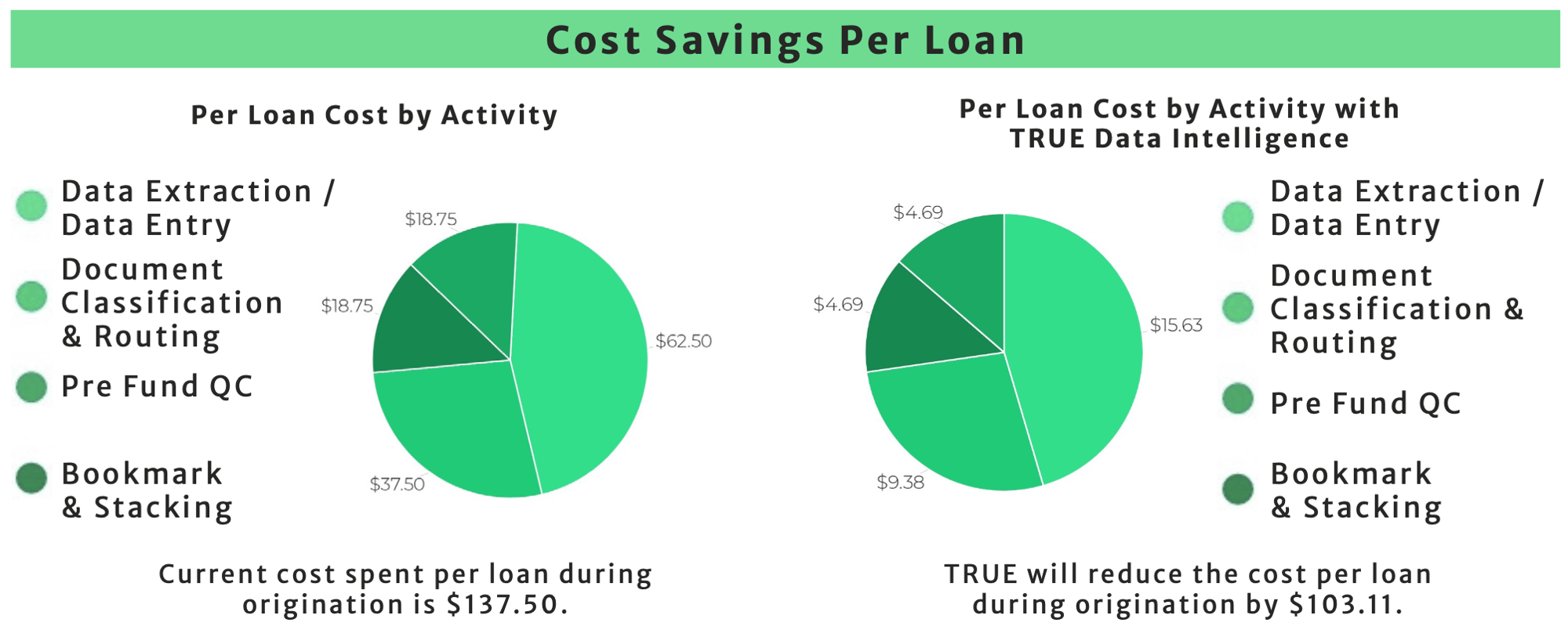
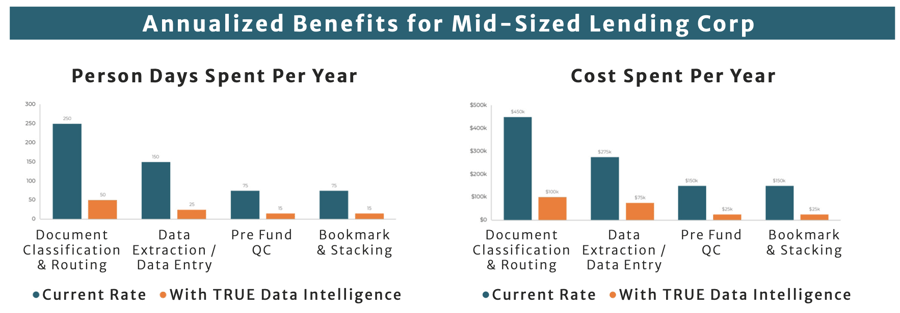
Part 3: Exponential Potential
As we established earlier, high quality data provides the building blocks for better processes. When the data is proven to be clean, it becomes trusted data. The combination of data quality and trust enables a tremendous range of additional use cases – a powerful multiplier of clean data ROI for business. Trusted data ROI can grow exponentially. It begets powerful analytical capabilities, new product development, closer customer and supply chain relationships, and value of the product portfolio based on it.
Our work with clients suggests that it takes very little time for most organizations to recognize where and how they can expand their use of clean and trusted mortgage loan data, because they can more easily see and understand so much more about their business. It is not unusual to see clients devising and implementing additional process and portfolio improvements within weeks of implementing effective clean data solutions.
Continuously Improving ROI
The ROI of clean, trusted data combined with intelligent automation grows exponentially as it builds on itself and enables transformation of business processes, especially in data-intense and highly- volatile financial operations.
For example, it is not unusual for clients properly implementing AI/ML- enabled process management software to realize first-year operational cost reductions of up to 30 percent. These cost improvements stem mainly from increased efficiency based on combinations of unified, clean, trusted data and intelligent automation that identify process issues while reducing errors and the problems associated with those errors.
ROI grows from there. In highly- regulated industries like financial services, we see client firms able to leverage their improved business and market intelligence to manage regulatory change more efficiently and rapidly than competitors.
But again, there’s more ROI to come. With mortgage processing automation, process efficiencies can continue to grow as markets change. We see clients with operational efficiencies improving as much as 5 percent to 10 percent annually for three or more years as their ability to use trusted data in more areas of the business expands and improves over time. The value and impact across the business is cumulative, as Figure 8 explains.
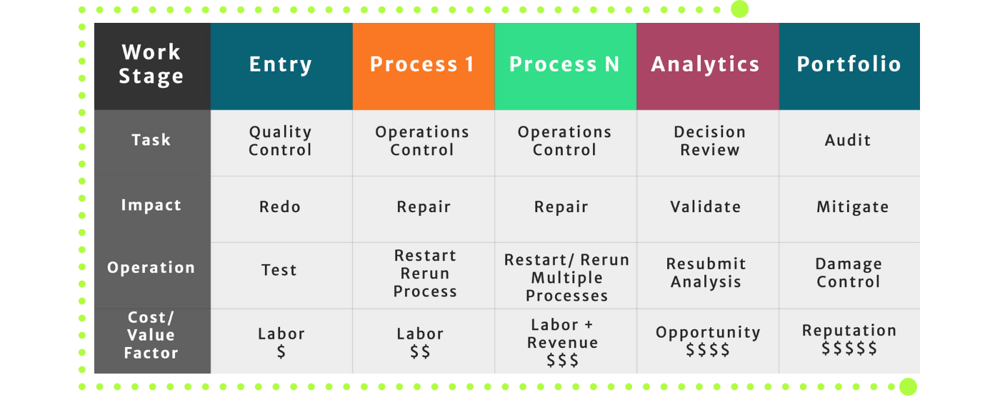
This extended ROI also includes the ability to create new lines of business – including product innovations or entry into new markets – at the lowest possible cost and with more accurate planning. As an example: a US-based financial institution client firm used cloud-based, intelligent automation
to clean and analyze transactional data to reduce data errors, handling costs, and process times, which improved its credit processing accuracy and efficiency while reducing customer and partner support costs.
Trusted data everywhere also enabled the client to explore previously unseen opportunities for new products, like credit and financing offerings tailored to individuals, affinity groups, and businesses by geography, profession, industry, and lifestyle. And it enabled new data products that were valuable to merchants and banks, to purchasing and spend management software and services providers, and to inventory and supply chain applications vendors and services providers.
9 Questions for Thinking About the ROI of Data
ROI sounds simple: will we get more out of this business investment than it costs? The reality is anything but. These questions help successful firms grow and thrive through trusted, clean, reusable data.
TRUE prepares you for what's next.
Our lending intelligence solutions make remarkably fast, transparent, and automated loan decisioning a reality — whether it’s for pre-funding or post-funding. Our team can work with you to assess your needs and objectives, and determine the best solutions for your business.