Executive Summary
Lending intelligence is set to define the future winners and losers in the mortgage industry. Information about buyers and the loan decisions this determines is contained in unstructured documents. Until recently, human skill was needed to read the documents and extract the relevant data. Today, artificial intelligence is outperforming humans at these tasks with greater accuracy and consistency. Using machines rather than people is resulted in trusted data, which lenders can use to transform business processes.
Key outcomes are gains in productivity, increased business elasticity to ever-changing volumes, and richer borrower insights that improve products and the customer experience.
Emerging from these changes is the realistic possibility of fulfilling the long-held goal of touchless automation – the ability to complete a process end-to-end with little or no human intervention – as advances in artificial intelligence enable humans to be taken out the loop for most data processing tasks related to mortgage origination.
As lending professionals know all too well, origination remains a highly manual process. Each application involves the conversion of borrowers’ documents into the data required by the origination process. The limitations of legacy data processing solutions mean that humans continue to be responsible for data classification, extraction, and multiple phases of data validation as mortgages progress through underwriting and approval, before yet more validation by the secondary market.
The result is a data bottleneck that puts limits on the speed and capacity of mortgage originators. Using human teams for data extraction and verification is expensive, and slow to scale to changes in demand. Consequently, processes are designed to extract only what is necessary for underwriting approval. This limits the scope of lenders’ data set, meaning valuable insights about borrowers are routinely overlooked.
The data bottleneck is both a threat and an opportunity for mortgage businesses. Human processing of data has remained steadfast in mortgage lending due to its document-intensive nature, but this makes the industry an outlier in an increasingly digital world. The lenders that will thrive in the future will be those who develop reliable automated processes that greatly reduce human intervention. These lenders will gain a scalable and cost-effective source of clean and trusted borrower data – lending intelligence – that will enable them to finally achieve the long-held goal of touchless automation.
The mortgage industry is experiencing the kind of digital that has fundamentally altered many other industries. While this transition has been taking place for several years it is accelerating now with next-generation AI-powered data automation technology.
In this new era, the industry’s winners will use lending intelligence and touchless automation to be more productive and efficient. Cost savings, reduced risk and a more elastic business model will translate into higher margins. A holistic understanding of borrowers will enable them to outsmart competitors. Lenders that persevere with business-as-usual solutions — including outsourcing or firing-and-rehiring of employees in response to industry and economic cycles — will struggle to keep pace against leaner, more profitable and better-informed rivals.
The Reality of Mortgage Industry Automation
Digital data has already transformed business models and the customer experience in many categories of lending, including auto loans, personal loans and credit cards. When all or most of your data is digital, processes can operate with little or no human intervention. This digitization in consumer credit has freed up markets, greatly expanding choice while significantly reducing underwriting times.
For example, most applications for credit cards can be completed online and approved in a few minutes. Some lenders will even provide a “virtual” card that works from your smartphone, enabling you to use your new line of credit instantly.
The mortgage industry has remained an island of continuity in this river of change. Loan applications may increasingly begin online, but behind the scenes are human-led processes that are counted not in minutes, or even hours, but days and weeks.
The Ubiquity of Documents
Mortgage origination is slowed down by the need for deeper level of analysis (due to the size of home loans and compliance with industry regulations) and the fact that information about borrowers is recorded in traditional documents: bank statements, paystubs, W-2 earnings, tax statements, personal identification, property appraisals, and more.
These documents may be physical paper or digital formats, such as PDFs, but either way the data they contain is unstructured, meaning it is not organized in a pre-defined manner that facilitates processing by computers. Borrower documents are typically text-heavy, have irregularities and ambiguities, and are received with varying degrees of quality. Correctly indexing mortgage documents and extracting the relevant facts, dates and numbers takes a trained and practiced eye.
The Quest for Automation
Automation remains one of the biggest gaps. In the hope of extracting some ROI, some lenders have added robotic process automation (RPA) to their LOS.
RPA offers a way to replicate human labor, using a set of pre-determined functions to perform highly consistent tasks. That’s useful when a task is reliably uniform, but that doesn’t apply to borrowers’ documents. The risk with RPA is that opportunities to spot errors are lost, while misinformation is created and accelerated through the origination process.
“RPAs are filling a gap,” explains Jennifer Fortier, Principal at STRATMOR Group, a specialist consulting firm for the mortgage industry. “This is functionality that the LOS should do but doesn’t.”

Loan Origination Systems
Mortgage lenders have invested in technologies intended to improve the speed and quality of origination processes and reduce the burden on lending professionals. At the core are loan origination systems (LOSs): platforms that structure and manage the entire process from application, through underwriting and post-closing.
LOSs have been in use for more than a decade, but they have largely failed to meet the industry’s hopes of an automated loan origination process.
“The industry got forced into this Frankenstein of piecing different systems together, and it’s hard to unwind it even though many are trying. There’s still no end-to-end solution; no panacea exists.”
- Kevin Peranio, Chief Lending Officer at PRMG
“Most lenders dislike their loan origination system,” says Rich Swerbinsky, formerly the President and Chief Operating Officer at The Mortgage Collaborative, a network of independent mortgage companies, banks, credit unions and mortgage service providers that work together to improve their businesses and the mortgage industry. “The vast majority of these platforms have failed to produce ROI [return on investment].”
The gap between expectations and experiences is due to the fact that, despite some vendor’s claims, LOSs offer little in the way of genuine automation. Rather, they’re tools that organize data for multiple stakeholders across the loan origination process. They may offer associated functions, such as the production of loan documentation, or interfacing with third party systems such as those for customer communications and compliance.
What an LOS cannot do – at least not with a sufficient degree of accuracy and consistency to be trusted – is generate the data they need to operate. Lending professionals remain responsible for loan delivery and everything associated with it – including those critical yet laborious docs-to-data conversion and verification tasks.
LOSs can also be hard to configure to the varying business models of different lenders. Unless your needs are unusually simple, most LOSs will need to be adapted. Lenders commonly develop their own configurations, add integrations, and even develop proprietary technology to get the functionality they need and which the core LOS doesn’t adequately provide. This practice is so well established that some LOS vendors support customization with dedicated marketplaces offering approved integrations.
While there is a lot of frustration with the costs and limitations of LOSs, lenders have invested money, time and have integrated their LOS into the fabric of their business. Moreover, they lack compelling alternatives.
“The industry got forced into this Frankenstein of piecing different system together, and it’s hard to unwind it even though many are trying,” says Kevin Peranio, Chief Lending Officer at PRMG, a residential mortgage lender. “There’s still no end-to-end solution; no panacea exists.”
What is Touchless Automation?
Touchless automation, also known as straight through processing, is the ability to use technology to facilitate a process end-to-end with zero human intervention. Software vendors have long promised the mortgage industry touchless automation. But over the years, lenders have learned that most vendors’ definition of “automation” includes a great deal of human intervention. In large part, this is because the mortgage industry is drowning in documentation and many of those documents contain troves of unstructured data that is critical to lending decisions. As a result, manual tasks have persisted and technology investments have failed to return the promised ROI.
The Data Bottleneck: What It is and Why It Matters
In mortgage industry jargon, origination is often known as “loan manufacturing.” The analogy makes sense: building a mortgage can be compared to the way automobile manufacturers construct cars.
Mortgages emerge from a production line where loans are pieced together from a series of data components. Like cars, there are standard designs (mortgage products) with customizations (programs, pricing, rates and associated services). Also, like car making, fixed costs are high and it can be tricky to match production volumes with changing demand.
Mortgage manufacturing volumes move through relatively predictable annual cycles made complex by irregular and less predictable factors: changes in the economy, fluctuating consumer sentiment, housing market trends, and market competition. These cycles and factors can combine to amplify peaks and troughs in volumes.
The result: lending professionals find themselves challenged to keep pace with demand in one quarter only to experience a lack of work during the next.
This is the mortgage industry’s perpetual challenge. Overburdened staff, stressed out by the demands of high volumes, are prone to making errors and this leads to increased risk. On the other hand, trained lending teams are a costly resource that cannot be left idle for long. Mortgage operations leaders build models to predict throughput and balance that against headcount, overtime, and outsourcing needs. Of course, no model is perfect and the impact is seen on the bottom line and in staff turnover figures.
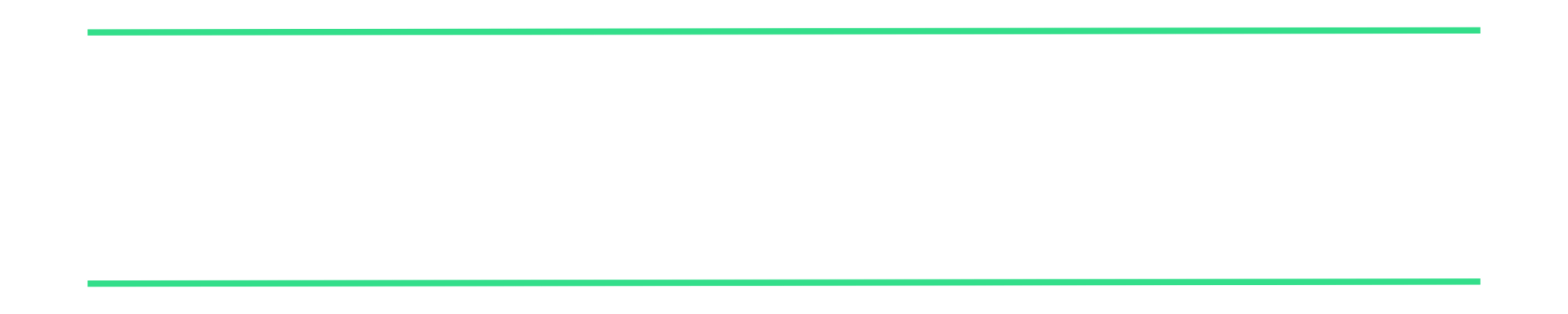
Inconsistent Components
There’s another challenge – and here’s where the manufacturing analogy breaks down. Unlike the parts used to make cars, the components needed to manufacture a mortgage are not identical. “So much of the origination process is unstructured and not uniform,” says Peranio. “Different pieces of data are collected at different times.”
Unlike car loan applications, where underwriting is based on readily available and highly structured digital data, such as credit scores, mortgage lenders need to analyze a combination of structured and unstructured data. The latter is mostly a mix of documents that vary not only in their content, layout and design, but also in their quality. Borrowers may fail to provide the requested document, can misdescribe what they provide, or take bad scans and blurry images.
For mortgage origination to move forward each document needs to be classified by the lender, any gaps or problems need to be rectified, and even once the application requirements are satisfied it will usually take several days to complete initial underwriting process.
“So much of the origination process is unstructured and not uniform. Different pieces of data are collected at different times.”
- Kevin Peranio
A Series of Bottlenecks
This is the first in a series of data bottlenecks. Lacking reliable automation, loan officers are engaged in a behind-the-scenes docs-to-data conversion task that is laborious, cumbersome, and error-prone. The first pass may be sufficient for initial underwriting, but as origination progresses the data must be verified and reverified several times. Each stage requires human intervention. This is vital and unavoidable to ensure accuracy and prevent avoidable risk in loan decisions, but these data bottlenecks repeatedly add time and expense to the origination process.
Throughput pressures mean operations teams focus only on the data that is absolutely needed. That’s reasonable given the circumstances, but a wealth of information about borrowers – data that is completely proprietary to a lender – is not captured. There simply isn’t enough time to extract every piece of data from countless pages of documents without making the process unworkable. The consequence is that a potential goldmine of borrower data is left unexploited.
The business outcomes for lenders include suboptimal lending decisions, where richer data could have improved the basis for declines and approvals, hidden risk due to human error and a lack of data sources for comparison, and a lack of innovation due to product teams being stymied by an incomplete picture of their borrowers.

Cost to Correct
The further erroneous data makes it into the origination process, the costlier it becomes to correct. “Vendors often don’t understand the implications of missing one small piece of data,” says Fortier.
If a loan officer assistant earning $20 an hour makes a mistake, that $20 problem will multiply into a $100 problem when later fixed by a higher salaried underwriter. If that error makes it all the way to closing, much of origination process needs to be rerun and the cost multiplies to $1,000 or more.
Errors directly translate into lost margin. Thier frequency depends on a range of factors, but whatever the average percentage of errors in initial docs-to-data conversion, calculating the true cost means multiplying it by the volume of applications and the rising cost of the corrective actions. It’s easy to see why even a small number of errors takes a big bite out of the bottom line.
Unfortunately, data bottlenecks don’t just hamper the origination process. They also slow down handover to the secondary market.
“Poor data quality is a trust issue,” says Rick Hill, Vice President of Information Technology at the Mortgage Bankers Association, the leading industry body representing all segments of the real estate finance industry. “Every entity that touches data needs to revalidate it along the way because they don’t trust what they get from the party who gave it to them. To me, that’s a big part of the cost.”
Untrusted data makes it harder to bring loan portfolios to the secondary market quickly, safely and cost-effectively. Verification processes by funders and servicers add another layer of delay, but are unavoidable because no party wants to be responsible for bad debts. If errors in a loan make their way into loan portfolios and the secondary market, and if that borrower defaults on their payments, the mortgage lender may be forced into a loan buyback.
Mortgage buybacks, also known as putbacks, can be ruinous for lenders. The costs are irrecoverable and the risk to the lender exists irrespective of whether it is a loan officer making a mistake or a borrower committing fraud.
“It has to be accurate data, or else the lender could be forced to buy that loan back,” says Swerbinsky.
“Poor data quality is a trust issue. Every entity that touches data needs to revalidate it along the way because they don’t trust what they get from the party who gave it to them. To me, that’s a big part of the cost.”
- Rick Hill, Vice President of Information Technology at the Mortgage Bankers Association
How to Solve the Data Bottleneck
So long as mortgage origination remains a manual process, headcount will continue to be the biggest determiner of throughput and operational costs. Predictive models and productivity tools can take the edges off peaks and troughs in demand, but fundamentally the size of the team matters.
So too does skill. Mortgage industry employees aren’t plug-and-play. According to Fortier, it can take as long as two years for a new hire in processing or underwriting to become fully proficient. It simply isn’t possible to continually adjust internal headcount without affecting the level of risk on loan portfolios or maintain a consistent quality of customer service.

The Hunt for Headcount
Many lenders have sought to increase elasticity in their business model through business process outsourcing (BPO). Contracting out some or all origination tasks to BPO providers can make it easier to align demand and supply, but it is not a complete solution.
When lenders select a BPO provider within North America, commercial, legal and cultural similarities make it easier to harmonize service standards. However, since lenders and BPO providers are recruiting in the same labor market, costs savings are unlikely and additional capacity may not be instantly available. If outsourcing is not flexible or affordable, its core value is undermined.
Offshore outsourcing, with the promise of reduced labor rates in developing economies, has been an attractive alternative for some lenders. However, as these economies mature local pay has edged upwards and cost savings have diminished. As this trend continues, lenders find that the savings are not worth the additional risks. Offshore BPO providers can struggle with customer service quality, that can harm a lender’s brand. Data security is threatened as customer information is transferred to different jurisdictions and entrusted to unknown workers using external IT networks.
So long as mortgage origination remains a manual process, headcount will continue to be the biggest determiner of throughput and operational costs.
People Don't Scale
The problems associated with a lack of elasticity were greatly exacerbated by the pandemic. Normal volume cycles were smashed by unprecedented shifts in demand as homebuying fell due to shelter-in-place orders, swelled due to changing consumer sentiment and work-from-home possibilities, then fell again due to rising inflation and interest rates. While 2021 saw a record $4.4 trillion in mortage origination, home sales fell 34 percent between December 2021 and December 2022.
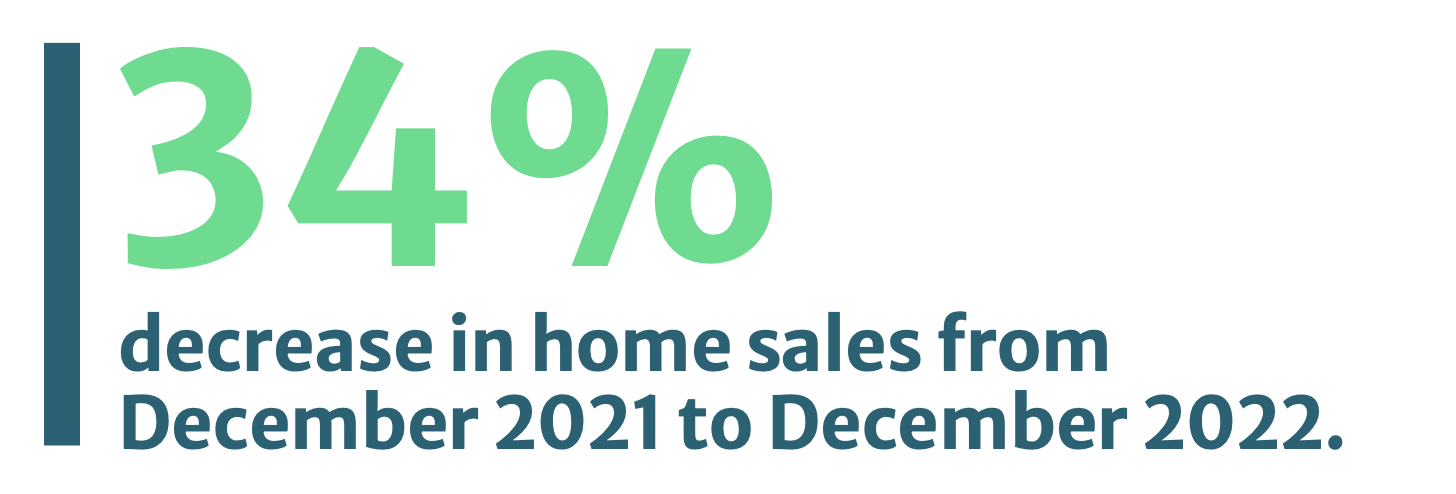
“I think decision makers are of the mindset that ‘I can’t continue to add and subtract human bodies like this anymore. One, it’s inefficient. Two, there’s the human side of it, unless you’re a cold-hearted killer.”
For this reason, solving the data bottleneck by adding people in the good times and letting them go when volumes subside no longer looks sustainable. “People don’t scale,” Hill points out. “That’s where some of the issues were in the last couple of years with the volume. In the perfect world, you’d just let the volume go up if the process is automated.”
A New Era for Automation
If hiring internally or outsourcing isn’t a good solution to the data bottleneck, what is?
Despite ROI disappointments with LOSs, lenders are continuing to look to technology as the solution to the lack of elasticity in their business model.
According to Swerbinsky, lenders that learned from the recent market turmoil are currently figuring out a technology-driven approach to loan manufacturing that’s faster, more efficient, and more cost-effective.
Developments in AI and machine learning (ML) are making it viable to automate docs-to-data conversion and verification tasks. When allied with LOS and point of sale (POS) systems, lenders can plot a course to a technology stack that enables their business to rapidly scale up and down as volumes change – without needing to adjust headcount. In this way, lenders can shatter the status quo of endless adaptation to cycling volumes.
Key to this is overcoming the questionable data quality of early data automation solutions. Lack of trust in the automation capabilities of LOSs has led to a costly and complex blending of humans and tech. Next generation systems with the self-improving capabilities of ML are able to reliably deliver clean data that lending professionals can trust.
It’s not easy for people to trust machines with business-critical tasks, but technology that delivers consistently high performance eventually proves itself. AI is leading the mortgage industry to re-evaluate automation and see if it really can eliminate the data bottleneck.
“The smartest lenders in America are looking really aggressively at this now,” Swerbinsky adds.
"We've seen the extremes these last three years...I would estimate 40 percent of the employees that were in the mortgage industry a year ago are now gone."
-Rich Swerbinsky, former President & COO at The Mortgage Collaborative
Automation Technologies for Borrowers' Documents and Data
Mortgage lenders have a choice of technologies that can help with the processing of borrowers’ documents, extracting the data they contain. The key ones are RPA and optical character recognition (OCR).
Both promise to bring efficiencies to mortgage operations and help lenders increase productivity. As lenders have learned from their LOS experiences, promises about automation should not be taken at face value.
RPA can be helpful in certain instances, but it’s not the basis of a long-term automation strategy, according to Fortier. “RPA just mimics what users do,” she Fortier. “It doesn’t really solve or even get into the space of ‘is my data good?’ Smart lenders recognize that and use it as a band-aid until they can get something better.”
While RPA will perform the same tasks consistently, lenders understand that without clean data there is a risk of errors being propagated faster. Human intervention will always be needed to monitor and correct this approach.
Similarly, OCR tools, which “read” imaged documents and extract text and numbers into predefined data fields, are notoriously unreliable. They are thrown by unfamiliar document designs, poor quality (blurred, misaligned) scans, variations in fonts, and even visually similar printed characters like 8 and B, 0 and O, and 1 and I.
Many lenders still opt to use OCR to reduce the workload for lending teams, but manual verification of all data is still required to ensure it is sufficiently cleaned. Some efficiency and productivity improvements can come from this approach, but duplicating tasks across machine and human severely limits the potential for improving efficiency and productivity.
True automation of docs-to-data conversion and verification tasks can only be realized when rates of data accuracy consistently match or exceed that of trained and experienced humans. That is now achievable as the iterative learning capabilities of AI are being combined with OCR to reliably deliver the level of data quality the mortgage industry needs if it is to take humans out of the loop.
"The big problem is the quality of data - being able to count on it and be 100 percent confident in it."
-Rich Swerbinsky
What Do We Mean by Clean Data?
As the old saying tells us: garbage in, garbage out. Clean data is the opposite: it is accurate, complete, and entirely trustworthy.
For mortgage companies, poor quality data adds risk to lending decisions. Errors have a growing financial impact the further they progress through the origination process. Should an error make it all the way to closing, lenders can be exposed to significant financial costs.
Lenders put significant resources into producing clean data, but there are limits to what can be achieved with manual processes.
TRUE: Lending Intelligence for the Mortgage Industry
TRUE is a first-of-its-kind platform designed specifically for the business needs of the mortgage industry. It supports a suite of highly configurable and dynamic AI-powered solutions that optimize data at every step of the lending automation lifecycle.
OCR tools for reading borrowers’ documents have struggled to match the accuracy of trained lending professionals, but TRUE significantly and consistently outperforms humans in docs-to-data conversion and data verification tasks.
Put another way, TRUE provides proven and reliable automation of the tasks that currently create the data bottleneck. As mortgage lenders deploy TRUE and gain confidence in its performance, trust in automated data quickly grows to the point where routine human intervention can confidently be withdrawn.
Eliminating most or all human intervention means the data bottleneck disappears and lenders can make a big step towards touchless automation of the entire loan origination process.
Why TRUE is Different
The TRUE platform uses OCR, but differs from conventional systems in two crucial ways:
Contextual analytics allows TRUE to go beyond classification and extraction, with a humanlike ability to compare data across multiple sources. This mimics routine yet sophisticated tasks, such as stare-and-compare analyses of income and spending.
These capabilities are pre-trained in the TRUE platform, making it the only solution capable of delivering accuracy rates of 95 percent out-of-the-box. Market-leading accuracy and the confidence this induces in system users are what define TRUE as a source of lending intelligence.
Increasing Accuracy Over Time
Human data classification and extraction accuracy rates in the mortgage industry vary by lender, but typically average 80 percent during the initial phase of the origination process. This is a lower rate than simpler data processing tasks in some other industries, but mortgage origination provides multiple opportunities for errors.
- Borrower data arrives unstructured and must be extracted from physical documents.
- The layout, design and details of documents is inconsistent and sometimes unclear.
- Document quality can be poor due to badly aligned, blurred or marked scans.
- Higher volumes create pressure to process more documents at the same time.
-
Pressure leads to errors, such as selecting data from the wrong document or the wrong version of a document.
These factors, combined with normal error rates for human data extraction and entry, make it virtually impossible to trust data quality. The only way to address this and reduce risk in lending decisions is to repeatedly verify the data at multiple stages of the origination process and again when loan portfolios enter the secondary market.
While the TRUE platform is more accurate than humans from the outset, typically starting around 95 percent, this quickly rises past 99 percent as its AI analytics engines are trained with a mortgage lender’s proprietary processes and customer data. The more loans TRUE solutions process, the more the underlying AI platform can learn. This makes lending intelligence a strategy for ever-improving quality and business performance.
These factors, combined with normal error rates for human data extraction and entry, make it virtually impossible to trust data quality. The only way to address this and reduce risk in lending decisions is to repeatedly verify the data at multiple stages of the origination process and again when loan portfolios enter the secondary market.
While the TRUE platform is more accurate than humans from the outset, typically starting around 95 percent, this quickly rises past 99 percent as its AI analytics engines are trained with a mortgage lender’s proprietary processes and customer data. The more loans TRUE solutions process, the more the underlying AI platform can learn. This makes lending intelligence a strategy for ever-improving quality and business performance.
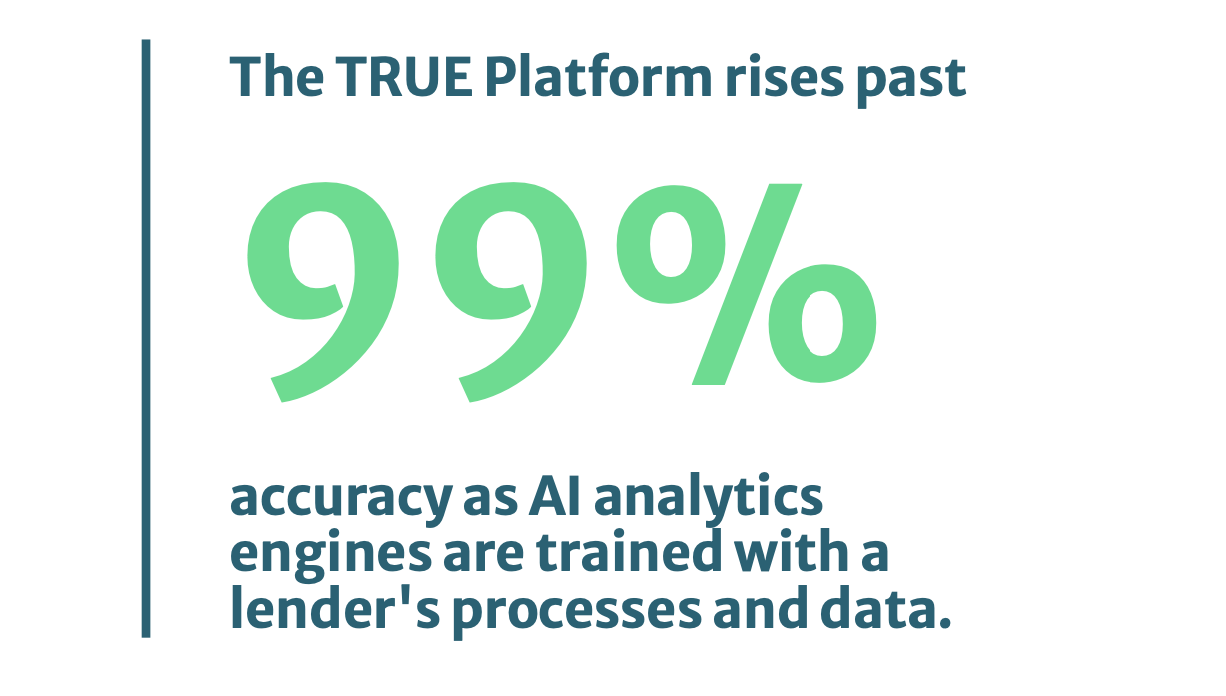
The only way to reduce risk in lending decisions is to repeatedly verify the data at multiple stages of the origination process and again when loan portfolios enter the secondary market.
TRUE Lending Intelligence Products
TRUE’s technology is purpose-built to help mortgage companies process and analyze documents with lightning-fast speed and unprecedented accuracy, regardless of volume,
The TRUE platform provides a complete lending intelligence strategy, based on two primary products:
TRUE Data Intelligence
TRUE Data Verification
4 Ways TRUE Delivers Lending Intelligence
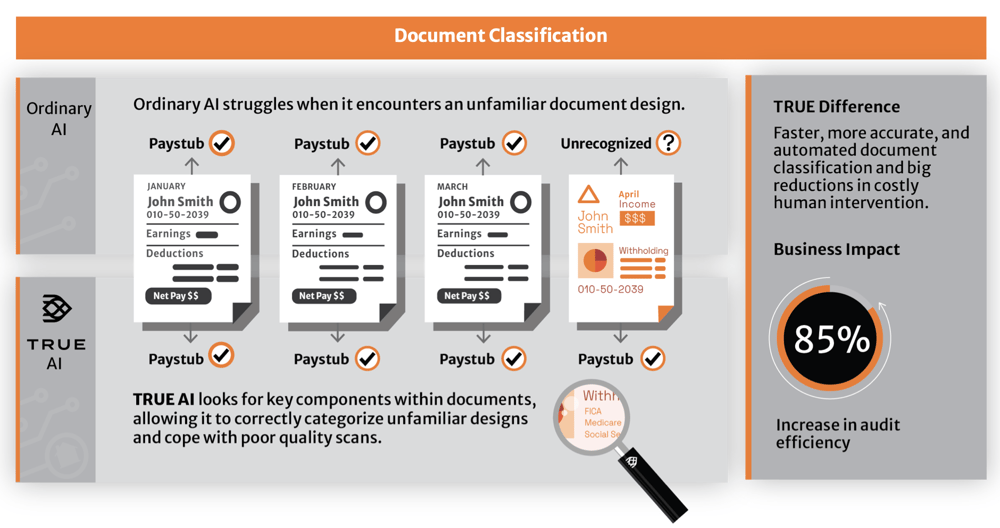
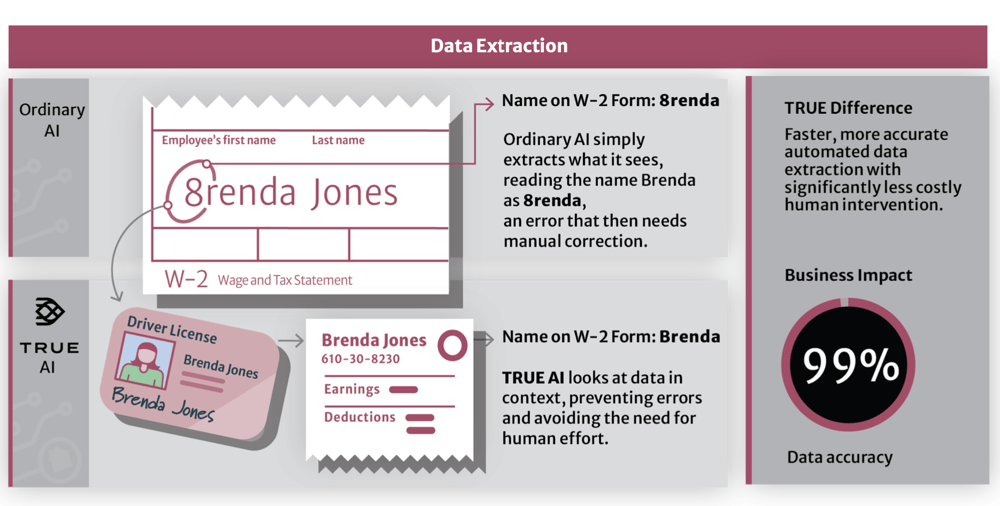
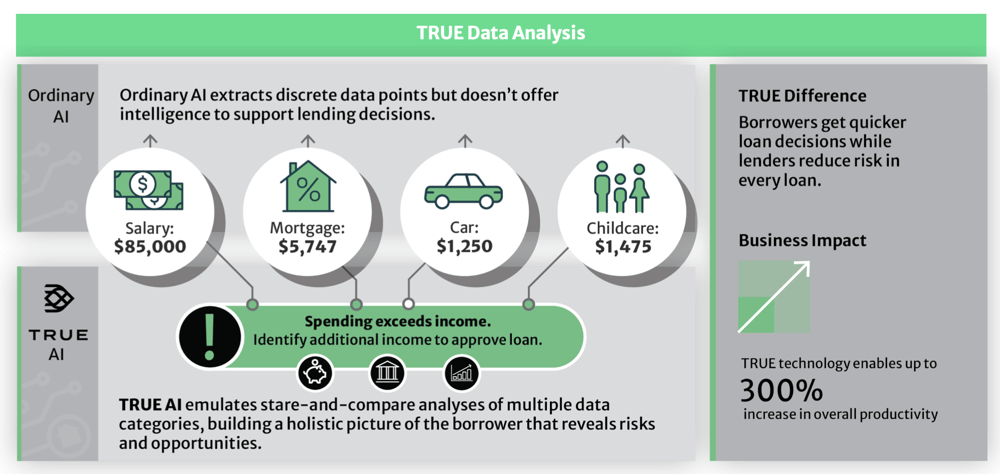
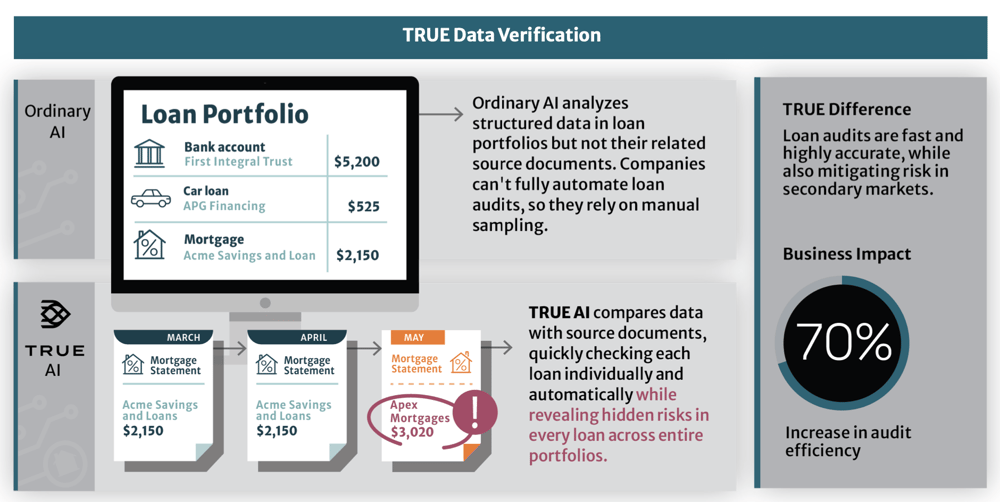
Lending Intelligence Transforms Mortgage Operations
Lending intelligence is the enabling link in a chain of automated process that can collectively be described as “touchless automation.” Different mortgage lenders will have their own business models and approach, but TRUE lending intelligence configures, adapts, and learns. It allows any lending business to develop a lending intelligence strategy and advance touchless automation.
Fortier describes touchless automation as: “completing a task, from trigger to conclusion, without human intervention.”
“I am starting to see real automation being offered,” she adds. “This newer functionality is beginning to focus on analyzing data, which is a considerable improvement in [the mortgage industry’s] ability to enable a truly exception-based workflow – where humans only needed to focus on things that need attention.”
As specific and then streams of tasks become fully automated, lenders can optimize staffing for service speed and quality. Defended against seasonality and economic cycles, resources can be released and deployed to improve margin, competitiveness, and the customer experience.
“Once you truly get there, it’s a complete game-changer,” Swerbinsky says of touchless automation. “It’s game-changing because a big percentage of loan manufacturing labor costs are in processing and underwriting. There are a lot of bodies in that phase. If you could eliminate that, if the data is sound, it reduces your risk for human error and fraud and things that would make you have to buy a loan back.”
Here are 6 possibilities with Lending Intelligence:
Streamline & Standardize Applications
The application process is the beginning of a series of interactions between borrower and lender that adds up to the “customer experience.” Alignment between sales claims and reality will be tested; brand ratings will rise or fall.
“If you work with processors and underwriters, you’ll see that how they think and approach what they do is just so drastically different from one person to the next, and it’s a big problem for lenders,” says Fortier. “Adopting some consistency is really, really critical.”
AI-enabled automation of document classification and data extraction tasks ensures that initial underwriting is handled consistently, improving speed and quality at this stage of origination.
The TRUE Data Intelligence solution can also be paired with POS systems to check that a complete and correct set of documents has been provided. Gaps or errors are spotted in minutes, helping lenders to direct digital or human support at this crucial first stage.
Strengthen the Customer Experience
According to a McKinsey report, 42 to 67 percent of borrowers said they were not satisfied with their mortgage process (with banks performing worse compared to non-bank lenders).
Starting well, with streamlined and standardized applications, is important. However, there will always be exceptions and edge cases. Some customers may have problems with their documents, or they may need specialist advice. The challenge for lenders is the time taken to handle exceptions, so a wise strategy is to use time savings from automation to redeploy staff.
“Getting that time back allows for exception processing on files that need a lot of manual hand-holding no matter what,” Peranio says. “Now, everybody gets more time to help that borrower, who oftentimes is a credit-challenged or even credit-invisible borrower.”
Satisfaction means more than the lowest price, but rising interest rates put pressure on lenders to be as competitive on rates and fees as possible. “When loans get cheaper, the consumer gets better rates, and everybody wins,” Peranio adds.
Unlock Productivity
TRUE solutions dramatically increase productivity across data classification, extraction and verification tasks, but lending intelligence unlocks new productivity possibilities. As the AI processes documents, a clean and trusted data resource emerges. This allows managers to more fully exploit the productivity potential of existing investments, including LOSs and RPA.
Lending intelligence can also improve the quality of work and employee satisfaction. “Would you rather be processing individual files or reviewing reports of hundreds of processed files?” Swerbinsky asks. “Anybody would prefer the latter.”
In addition to doing higher-value, less tedious work, lending professionals can use the time they win back to add value to the business. That includes optimization of business processes, increasing sales, and providing human support to customers when that is required.
Create an Elastic Business Model
An end to the perpetual challenge of matching staffing with volumes is a dream for operations leaders, but lending intelligence can finally make this happen.
Volumes will continue to peak and trough, but automation of labor-intensive processes prevents lenders from having to add and subtract headcount or outsource work to BPO providers in response to shifting market conditions.
TRUE enables lenders to build truly elastic business models from the moment of deployment. Fees are set per-funded-loan, so costs match capacity as lenders scale up or down. In addition to costs, profit margins and service standards become more consistent and predictable.
Accelerate Product Innovation
“It’s not just the quality of the data, it’s how much is even collected,” Peranio says.
Unlike manual data extraction, there is no limit to what data can be extracted from documents through AI-enabled automation. That allows lenders to gain proprietary data insights that give a more holistic view of the customer, enabling optimization of existing products, and prompting innovation.
Consider trends in work. We may have a general sense that more people are active in the gig economy, have more than one job, or independent side hustles. Lending intelligence will reveal insights from the data describing these trends, enabling product teams to better serve a changing market.
For example, a decade ago lenders might have applied more scrutiny to 1099 contractors because their income wasn’t as predictable as their W-2-only counterparts.
Lending intelligence could show hidden strengths in earning patterns leading to more contractors being approved — a major change from years past. Understanding this, product teams might decide to take a data-driven approach and create mortgage products designed for borrowers who work 1099 jobs. With TRUE extracting all data from every application, this kind of innovation becomes much easier and faster.
Gain Confidence in the Secondary Market
Last, but very far from least, lending intelligence increases confidence in loan quality between originators and the servicers and financers in the secondary market. TRUE solutions reduce defect rates to the lowest possible level – just fractions of a percent compared with 10 to 15 percent error rates in the early stages of manual processes. “At the end of the day, the secondary market is driving all of the hesitation about data,” says Swerbinsky.
Mortgage buybacks or putbacks are a huge loss for any lender and can be existential for some providers. “Independent mortgage bankers have had a tough year, most have lost money, so the impact of having to buy loans back is greater,” says Swerbinsky.
TRUE Data Verification gives originators complete confidence in the completeness and accuracy of data in their loan portfolios, significantly reducing the risk of buybacks. Secondary market organizations can use the same solution to switch auditing of incoming portfolios from a sample to as much as 100 percent of loans without needing any additional time. Deals close quicker and loans are securitized faster, further reducing market risk.
“The thought that ‘wait a minute, my data doesn't equal my docs’ kind of goes away. I'm already hearing from folks that they're seeing several hundred dollars per loan alone improvement,” says Hill.
Window of Opportunity
These factors, combined with normal error rates for human data extraction and entry, make it virtually impossible to trust data quality. The only way to address this and reduce risk in lending decisions is to repeatedly verify the data at multiple stages of the origination process and again when loan portfolios enter the secondary market.
While the TRUE platform is more accurate than humans from the outset, typically starting around 95 percent, this quickly rises past 99 percent as its AI analytics engines are trained with a mortgage lender’s proprietary processes and customer data. The more loans TRUE solutions process, the more the underlying AI platform can learn. This makes lending intelligence a strategy for ever-improving quality and business performance.
United States Average Hourly Wages
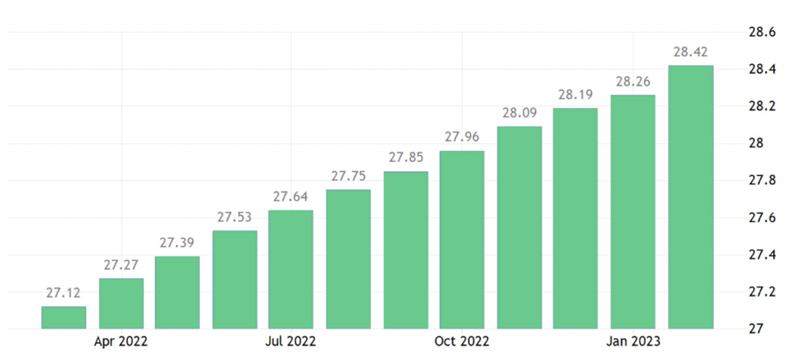

Digital Disruption
As some lenders adopt new solutions to eliminate the data bottleneck and look to automation to drive efficiency, productivity, elasticity, and competitiveness, others will maintain business-as-usual practices. This could become a challenge as more lenders digitalize their data processes, with AI making it possible to include what was previously unstructured data.
Mortgage providers that operate with fully structured data can develop products and business models that deliver a customer experience more akin to credit card, personal loan, or auto lending. While differing regulations between mortgages and other categories of lending mean that a direct comparison should not be made, the foundations are being laid for an era of digital disruption.
Disrupted industries look different, with new business models leading to changing fortunes for established firms and the emergence of new competitors built for the new era. This has not happened in the mortgage industry yet, but lending intelligence may prove to be not just an enabler but also a catalyst of change.
Experience TRUE Lending Intelligence
The mortgage industry runs on data, but difficulties with completeness and accuracy have made that data impossible to trust. A complex and risk-prone blend of human skill and technology is accepted as the cost of doing business. Artificial intelligence is removing these challenges… forever. Next-generation optical AI, built by TRUE, reliably outperforms humans at reading, classifying, and extracting data from borrowers’ documents. The result is trusted lending intelligence. The impact of trust in data is genuine automation in origination and servicing – something that the mortgage industry has been seeking to achieve for many years.
Further Reading
Market trends, industry reports, customer case studies and AI insights to help you navigate digital transformation, innovation and next-gen mortgage technologies.
Download full report
Click through to download our full Lending Intelligence Whitepaper in a PDF version.