From Artificial Intelligence to Lending Intelligence
The inner workings of AI that is transforming mission-critical data used by every stage of the mortgage industry.
Why AI? Why Now?
AI has advanced considerably in recent years. The latest systems can reliably perform complex tasks that until recently we thought of as intrinsically human: writing, illustrating, driving a car, even delicate surgery.
I’ve studied and worked in AI since I began my PhD in computer vision in the mid-1980s. Since then, I’ve published over 40 papers and have gained several patents in areas related to imaging, machine learning and automation. I’ve applied my research in businesses offering image compression, optical character recognition (OCR) and AI-enhanced business process automation (BPO).
AI tends to develop in waves, usually accompanied by media hype that can be quite effective for driving investment, applications and deployments. With each wave we’ve gained enhanced capabilities that make AI increasingly useful.
Contemporary AI systems are highly effective at solving specific problems and performing generic but well-defined tasks. They have become deeply rooted in many aspects of our lives: work, travel, entertainment, healthcare, and critical infrastructure including financial services. The value of integrating AI into operations and processes means that we’ve entered an era where it is usually a question of when, not if, AI will enhance, disrupt or reinvent a job or industry.
AI and the Mortgage Industry
My focus, and the subject of this paper, is a category of AI built exclusively for the mortgage industry. At TRUE, we build software that’s used by lenders, the secondary market, and most mortgage insurers. It largely solves what I and many esteemed industry commentators believe is one of our industry’s biggest problems: the high costs and operational bottlenecks of cleanly capturing data about borrowers.2
While some parts of the mortgage industry are taking advantage of advanced automation technology – notably mortgage insurers – many lending businesses and investors have not fully grasped the potential. The current AI hype, especially about generative AI systems, has been overwhelming and leaves many business leaders unsure about which AI investments make sense.
My aim is to demystify AI as it applies to the mortgage industry. I hope to offer insights that will be helpful to you, as an industry leader and stakeholder, when you evaluate AI and consider solutions that offer the greatest business impact and financial return.
Today’s AI systems are a product of how AI has developed over several decades, so I’ll provide the long view before setting out my position about where and why the latest AI technology can help to resolve mortgage industry challenges and accelerate loan decisioning. I’ll then explain, in plain English, how the AI we’ve built at TRUE works.
The outcomes we deliver not only involve complex data, but also human emotions and a very high cost of errors (in reputation and costs for lenders and in life impact for borrowers). With this in mind, I’ll explain how those using our AI learn to trust the technology.
Finally, given the public debate about the rewards and harms associated with AI, I will touch on the impact for mortgage businesses and jobs. I hope to leave you with a greater appreciation of why AI, built specifically for mortgage lending, is a welcome change that can enhance productivity, opportunity and the customer experience without threatening the sustainability of our industry and the employment it provides.
The Story of AI and How to Trust It
How many articles about AI have you read that are illustrated with some sort of android… or even The Terminator? Putting benign or malevolent intentions aside, these representations suggest not AI but an artificial general intelligence (AGI) – a hypothetical technology capable of mastering any intellectual task that can be performed by a human.
AGI has not yet been achieved and, depending on who you talk to, it is somewhere between decades and forever out of reach.
It all started with the development of electronic computers in the 1940s-1950s. AGI was how AI was originally envisaged.
Pioneers like Alan Turing proposed standards for machines capable of simulating human intelligence. The term “artificial intelligence” was coined at a workshop at Dartmouth College, New Hampshire, in 1956.
Early progress led some to believe that human-level AI was just around the corner, but researchers found that many reasoning tasks were more complex than initially thought. The limitations of the era’s computers became increasingly evident. A period known as the “AI Winter” followed as interest waned and funding dwindled.
By the 1980s, a resurgence of sorts came in the form of “expert systems.”
A rules-based “if this, then that” form of AI was created.
More powerful computers and the accumulation of digital data led to a resurgence of interest and investment in AI. As the new millennium began, machine learning (ML) techniques were bringing new capabilities and expanding the practical applications.
By the 2010s, AI was being experienced in daily life.
Think of the personalized product recommendations that are part of shopping online, virtual assistants such as Siri or Alexa, or facial recognition tools that help to organize your photos.
So what now?
The latest development is generative AI, which takes advantage of today’s vast datasets and computer speeds to build digital neural networks capable of generating content based on the patterns it observes in other content. If you’ve tried some of these tools (famously ChatGPT, but there are many others producing text, images, videos, audio, 3D models, and more) the results can be so impressive that you might think that we’ve achieved AGI.
In fact, what we experience is the result of progressive iterations of so-called “weak AI,” also known as “narrow AI,” which focuses on performing a specific task. This contrasts with “strong AI,” the human-like AGI that was envisaged in the 1950s. Labeling AI as “strong” or “weak” suggests systems that are more effective or less effective, but in this context the terms describe the approach taken to building and operating an AI rather than its ability to perform. So-called “strong AI” does not yet exist, whereas “weak AI” based on iterative training using massive amounts of data is anything but weak. An alternative definition is “narrow AI,” though better still, in my view, would be something like “focused AI.”
So-called “weak AI” or “narrow AI” lies behind the transformative machines that not only match but greatly exceed human skills at specific tasks. Whatever we want to call it, the results of this approach are truly impressive: software that can drive a car, paint in the style of any artist, diagnose lung cancer, optimize transport networks, or fly military jets.
Trust me, I'm a machine
What does it take for us to trust a machine?
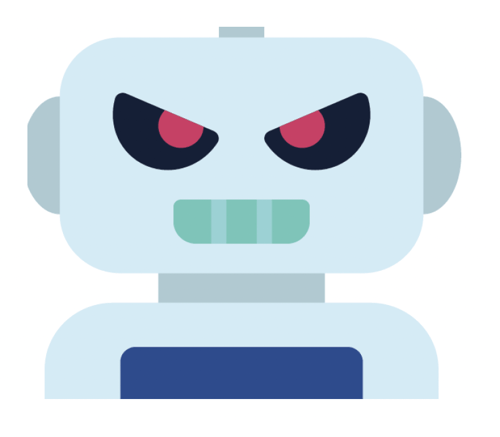
AI used to supplement or replace human labor and intellect should be able to work effectively, consistently and safely. However, the confidence we need to trust machines varies considerably with the type of work we give them.
I feel quite differently about the AI that handles the autonomous driving functions in my car than the AI that manages the cleaning cycles of my washing machine. The risks involved are very, very different!
Homebuying doesn’t involve physical risks, but it is one of life’s biggest and most consequential financial decisions.
Calculating transactions is straightforward, but for both lender and borrower the quality of the data used to determine the price and risk of that loan is paramount. For the homebuyer, an approval means getting the home they want. For the lender, flawed data risks an incorrect decision and a costly buyback.
The salient point here is that we have a level of tolerance for errors. When the stakes are low, our tolerance is moderate. When the stakes are high, we become highly intolerant of errors. It follows that when it comes to mortgages, any AI used to manufacture a loan and support a lending decision must be
highly accurate.
Is it Safe to Drive?
Humans and AI are both good at pattern recognition. The more frequently we are exposed to similar sets of data the greater our confidence in how to react when we next encounter similar data.
The challenge (for human and artificial intelligence) is discerning when the data has changed enough for us to question our confidence and proceed with renewed caution. Too much confidence and we risk making a mistake. Too much caution and we get nothing done.
Consider the following examples:
It’s relatively easy for us, for humans, to recognize the fundamental similarity of these situations. In the first three scenarios, there’s a crosswalk and pedestrians, but the road is clear and the traffic lights are green. The mix of pedestrians isn’t the same in each example, but that doesn’t change our assessment of the situation. It’s safe for us to continue driving. The last image looks a little different. Is it still safe to drive? The horses are an unusual sight, but we can see that they are waiting with some humans. The crosswalk is clear and the lights are green, so we can safely drive on, perhaps with a slower speed and increased caution.

Now, consider the following borrower documents. In this case, a series of paystubs. Again, we see that these are all different versions of the same type of information. In the first three documents, we can easily identify the data we care about: the name of the employee and employer, earnings, deductions and benefits, and the date. However, the final paystub looks different. Is this document still a paystub? The paystub has an unconventional layout - perhaps it was designed by an in-house accounts team - but we can see that it contains all the expected information that qualifies it as a paystub.

Is it safe to drive? Is the document a paystub? Can we identify the data we need?
The lesson of this illustration is that the more examples and variations of a data set we have, the better our ability to infer from that data. Humans and machines process data differently. An AI driving system would need to be trained with many examples of untypical situations to be able to act safely. Humans need fewer examples of a data set to be able to make sense of it.
We sense the world more richly than machines and we have an ability to generalize that is a huge strength – one that AI has been unable to match.
Although machines are poor at generalizing, they have endless patience for learning from examples. Machines can process billions of examples of similar data sets to learn how to interpret variations around a single, specific task. Once they’ve assimilated that data, they can become extremely good and very fast at performing that task.
My point here is that for human or machine our ability to perform an intellectual task – to make a decision or a prediction – is a matter of acquired knowledge (which is really just data) and confidence.
Confidence and Accuracy
OK, but what about that matter of tolerance? At some point we cross a line between unsure and sure. How does a machine determine this?
We think of computers as binary machines, dealing with 1s and 0s, yes or no, positive or negative. When we only have these two options, we can act with 100 percent confidence. Our accuracy is perfect. However, perfect accuracy is hard to achieve when you come out of the binary world and into the real one.
Let’s go back to our illustration. How confident are we that it’s safe to drive? It’s high, but probably not 100 percent. We know there’s always the possibility of a hidden danger, or a misinterpretation of the behavior of the pedestrians. What about an autonomous car deciding whether to stop or continue? Likewise, how confident are we that we’re looking at a paystub and not some other type of document? AI gives machines the ability to deal with more complex situations where the available data offers less than 100 percent confidence. We need to set a threshold for confidence at which we consider a data-driven decision to be accurate. It’s a judgement call – and an important one at that.
To help us think about this, let’s widen our perspective for a moment and think about the AI of the moment: ChatGPT.
As we established earlier, ChatGPT is great at imitating human creativity but, like all AI, it is “weak” or “narrow.” This is where that endless patience is a technological virtue. ChatGPT is what’s known as a large language model (or LLM). It’s been trained on the biggest language data set possible: billons of pages of text from across the web.
While the task is narrow, the application is broad. ChatGPT needs only simple instructions to perform an incredible variety of writing tasks – anything from writing recipes based on what’s left in the fridge to writing a travel guide in the style of D.H. Lawrence.
Many businesses are finding ways to use this capability to supplement human labor and intellect, but broad applicability comes with a cost: ChatGPT and other forms of generative AI score low for accuracy.
According to one study, with the latest ChatGPT-4 release, prime number identification accuracy fell dramatically from 97.6% in March 2023 to 2.4% in June 2023.7
Narrow AI tools with relatively broad applications are readily available and are frequently inexpensive (often free to the casual user and keenly priced for commercial users), but confidence tolerances are set low to make the tool useful to as many users as possible. That may be fine for some tasks, but remember how we feel about errors in financial transactions?
When our tolerance for errors is low, our confidence score, that line that separates unsure from sure, must be set high.
How high? In my view, the AI must be at least as accurate as a human who is an expert in the task. In fact, I’d argue that the accuracy of AI in mortgage lending needs to be considerably higher than a human.
One commercial reason is that you need to justify the change management of automating a task (change always involves a level of financial cost and organizational disruption). Far more important, in my experience, is that humans are considerably less tolerant of errors made by a machine than those made by a human.
Why? Because we’re human, not unthinking automatons. We make mistakes, we know everyone else does as well, so we can accept and adapt to the failures of others. We’re not so accepting of the soulless machine. It does just one thing, so it had better do it really well! Since it can’t answer back, it’s easily dismissed as inadequate or untrustworthy.
How do you build an AI solution that people can trust?
The answer is specificity. Focus the application and training so the AI is capable of very high confidence and therefore accuracy. When the AI significantly and reliably outperforms even the most capable of humans, that trust is earned.
Data Quality and the Data Bottleneck
What business problem would you most like to solve with AI? In a cyclical industry like lending, perhaps you’d like software that can more accurately predict volumes. Given recent challenges with recruitment and retention, maybe you’d like better tools for screening prospective employees and managing job satisfaction. Or, in an era where consumers are accustomed to instant service, could a genuinely smart chatbot be high on your list?
AI can help with all of these, but let me explain why I believe that data is your biggest problem and why AI-powered data automation will yield by far the greatest business impact.
Every financial services business runs on data. When all or most of that data is digital, business processes can operate with little or no human intervention. A key example from lending is the credit card industry. In 2022, U.S. credit card transactions totaled $54.8 billion. That works out at 1,739 transactions per second. The volume, speed and ease of these transactions is testament to the power of digital data to facilitate choice, quality and opportunity.
This phenomenon is not unique to credit cards. Other forms of lending, such as personal loans and auto loans, have also benefitted from thedigitization of customer data. In each case the shift to digital has seen markets grow, consumer choice expand, and underwriting times fall to just minutes for the majority of borrowers.
The mortgage industry has remained an island of continuity in this river of change. Loan applications may increasingly begin online, but behind the scenes are human-led processes that are counted not in minutes or even hours but days and weeks. Auto loans, personal loans and credit cards can all be underwritten swiftly and with minimal hassle. Why isn’t this the case for mortgage lending?
Documents and Unstructured Data
The simple answer is the format of the data. Many forms of consumer credit are underwritten purely on the basis of electronic data. We declare our financial circumstances through a form and these claims are verified by comparing them with data that the lender may already hold about us and trusted data from an independent third-party, typically a credit rating agency.
The key factor about these data sources is that they are structured and stored electronically. This allows verification processes to take place in mere seconds.
What do I mean when I say that data is structured? Here’s a definition from G2:
“Structured data is highly organized and formatted so that it’s easily searchable in relational databases. Unstructured data has no predefined format or organization, making it much more difficult to collect, process, and analyze. Structured data is more finite and sorted into data arrays, while unstructured data is scattered and variable.”
Some of the data we handle in mortgage lending is structured, but much of the most important data is not. The larger loan sizes involved in homebuying means lenders are quite rightly (and compelled by regulations to be) more cautious. We therefore look for evidence beyond a credit score to verify income and affordability. That evidence comes in the form of documents: paystubs, bank statements, W-2 forms, etc.
Data contained in these documents is unstructured. The pages must be read, understood and resolved against each other to enable specific and essential information to be extracted while irrelevant information can be safely ignored.
There’s more. Mortgages are secured loans, with the home as collateral. We also need information about the property as part of the risk assessment for each loan decision. That information also comes in the form of documents: property appraisals, surveys and contracts, property intelligence reports, etc. Again, everything needs to be read, understood and resolved to give us sufficient structured data to run the loan origination system (LOS) and make a decision to offer or deny the loan.
To those unfamiliar with mortgage lending, the prevalence of documents in our industry can seem almost archaic. And while it’s true that most documents are at least captured and transmitted electronically (usually as JPGs or PDFs uploaded via an online portal), we’re effectively receiving photographs of documents which still need to be understood visually.
Examining all these documents to obtain the data we need to manufacture a loan and assess risk is a complex, expert and time-consuming task. The initial document intake is the first in what ends up being a series of document- related data bottlenecks – data assembly and review processes that are obligatory yet always constrained by the speed, cost and capacity of human teams.
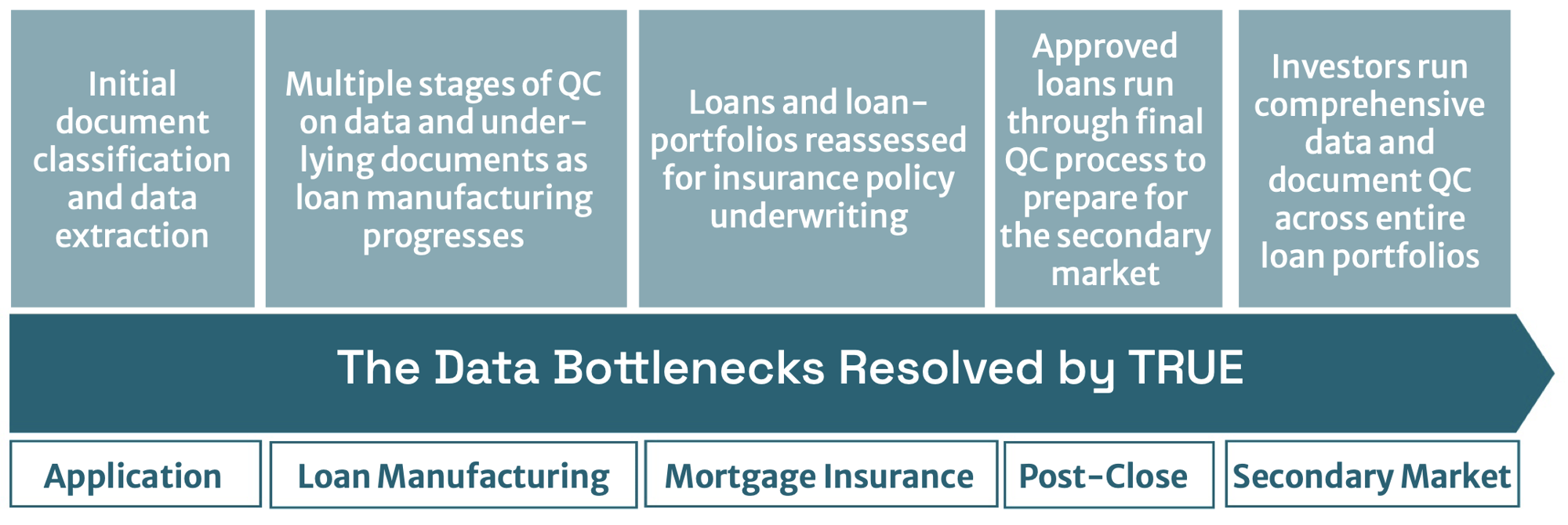
A Trail of Mistrust
Despite the investment that is made to capture accurate data at the earliest stage of loan origination, lending organizations do not trust it. Everyone along the origination chain understands that document reviews are laborious, detailed and prone to error. That first pass may be considered sufficient for initial underwriting, but as origination progresses lenders verify and reverify data multiple times.
Is the data complete?
Does it match the documents?
Do we have the latest versions of the documents?
Each of these quality control (QC) checks requires human intervention. These checks get more costly the further we get into the origination processes as more qualified, experienced and higher paid employees get involved. Even senior underwriters carry out their own QC checks because, despite knowing that multiple reviews have already taken place, it’s their reputation and job on the line if a bad decision is made.
It’s not just originators. Mortgage insurers conduct document and data reviews before pricing and underwriting policies.
Secondary market financers sample incoming loan packages to ensure a satisfactory level of loan quality.
While this layering of QC processes has a high cost, the cost of errors can be higher still. Defaulting borrowers are a cost risk for secondary market financers and insurers. However, if a defaulted loan can be traced
to flaws in the data (and therefore the documents), buyback clauses put responsibility on the originating lender. And we’re talking hundreds of thousands, even millions of dollars!
Machine Reading Documents
When you contemplate the scale and ubiquity of this document reading challenge, it’s easy to appreciate how valuable it would be to automate these tasks. A machine able to read, resolve and extract data from documents, with human-like skill, would reduce costs, time and risk for every originating lender, insurer and secondary market funder.
Machines that scan documents and capture text have existed for many years and even basic models often include software capable of this task. However, they are not nearly accurate enough for regulated industries, including mortgage lending. Remember, this is a financial transaction based on “scattered and variable” unstructured data and any automation technology would need to be reliably accurate.
But imagine what it would mean to have truly solved this problem. It would equip the mortgage industry with structured and trusted data resources more akin to those in other areas of consumer credit.
This leads not only to faster and lower-risk origination, but unlocking potential for product, service and pricing innovations that would be transformative for our businesses, borrowers and broader society.
This vision of an efficient, fast and flexible mortgage industry is not mine. Originating lenders have been chasing the goal of “touchless automation” or “straight through processing” (STP) for many years.10 The blocker was never the business processes, or the LOSs intended to streamline them, but instead the flawed quality and slow speed of incoming borrower data.
Machine reading of documents that surpasses the abilities of trained mortgage industry professionals has now been achieved. AI that can read documents – accurately and reliably – is making the STP vision possible in ways that were impossible just a few years ago.
Lending and Data Automation: What's the Difference?
Lending automation is the “Holy Grail” of the mortgage industry, but attempts to achieve it have floundered. When you look at other industries that are highly automated, from car manufacturing to payments, all have solved their data problem.
In fact, the lending automation piece is a rules-based system that would be relatively easy to achieve. The obstacle is the constraints on the supply of clean data due to manual processing. Clean data is data that is accurate, complete and entirely trustworthy.
Once you have clean data, without constraints, you then have the basis for data automation and the means to drive lending automation.
What is TRUE AI?
We’ve covered the history of AI and my argument for why AI-powered data automation is a priority for the mortgage industry. I’d now like to introduce the AI we’ve created at TRUE: a platform and solutions that are purpose-built to address the mortgage industry’s data problem. I’ll explain how our technology works and what you can do with it.
TRUE technology is based on a proprietary optical AI that transforms the unstructured information in borrowers’ documents into structured, rich and very clean data that can flexibility be used to originate mortgage loans and automate QC checks. We call it “lending intelligence” and it’s used to automate and optimize processes at every stage of the lending lifecycle.
-
It allows mortgage lenders to rapidly process loans, drastically cut costs and risk, and radically improve the customer experience.
-
It allows secondary market servicers and funders to rapidly assess the integrity of individual loans or entire loan portfolios.
-
It allows mortgage insurance providers to rapidly underwrite policies with more confident calculations of risk and more competitive pricing.
How Do We Create Lending Intelligence?
Step 1: Read and understand each individual document
Click to learn more
Documents are scanned or photographed so they can be read using OCR. The AI recognizes the document type, then extracts all relevant data. The result is structured borrower data that remains associated with the original document.
Step 2: Compare all data from all documents globally
Click to learn more
Cross-comparison of all data about the borrower and their loan is like stare-and- compare on steroids. It increases confidence in data accuracy, whileidentifying inconsistent or erroneous data for human attention.
Step 1: Learning to Read
How did we teach the TRUE AI system to read borrowers’ documents... and do so better than any other AI?
Let me offer another illustration. As humans, we learned how to read by being introduced to many examples of words, numbers and other symbols.
We became familiar with the shapes, being able to recognize and make sense of them even when they are presented to us in different fonts, sizes and colors.
The shapes of most letters and numbers are distinctive, but some are similar: 1s and ls, 0s and Os. Telling these apart takes a little more practice, but eventually we learn to tell the difference not only through shape but also through context.
It’s obvious to me that the “O” in the word “Obviously” is not a zero because a number would not normally appear within a passage of text. I know this from reading the word “obviously” in such a context hundreds, probably thousands of times.
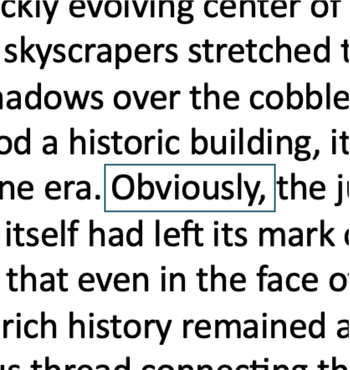
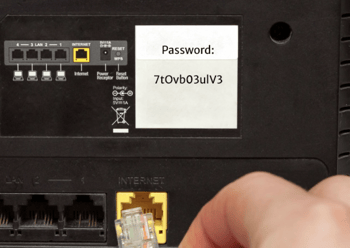
However, when the context changes we start to feel the limits of our confidence.
A WiFi password designed to enhance security will often mix upper- and lower-case letters with numbers in a random sequence. With the sequence “Hr76fF3OLl8”we may lack confidence that “O” or “l” are letters and not numbers, so we must return to what we know about the shapes of these characters. We can see that “O” and “0” are not quite the same shape and we understand the difference. With “I” and “l” the difference is more subtle, making comprehension even more challenging.
An AI system can face similar confusion, but it has some advantages and disadvantages compared with the human reader. The processing power and perfect memory of a computer enables the AI to learn huge libraries of fonts. The machine can discern the different shapes of characters down to the level of a single pixel.
The corollary is that an AI can struggle when it encounters an unfamiliar font or when the resolution is poor (such as grainy, blurred, pixelated or obscured characters). To solve for this, we can use context (as with “Obviously” above). Does the character appear to be part of a word? What other instances of the character can we read from that page, or from the entire document? The more we learn about the environment of a page and across multiple pages, the more confident we can be about recognizing a single character.
Is This Really Necessary?
You may be thinking, this is all very well but aren’t documents digital these days? Wouldn’t it be easier to process documents electronically rather than teaching a machine to read? There are a few of problems with this:
- A photo or scan of a document turns it into data that can be transmitted electronically but the information is still encoded visually.
- Although a “native” PDF (one that was created electronically in the first place) allows the OCR step to be skipped, in many cases we need to verify the visual presence of handwritten signatures and their associated dates.
- The association between the data and the underlying documents must be visual to enable a clear audit trail.
- The Equal Credit Opportunity Act (ECOA) and the Home Mortgage Disclosure Act (HMDA) require a visual observation of the borrower or a reading of their last name to collect monitoring information regarding age, sex, marital status, and race or national origin.
Step 2: Global Data Comparison
Once we know how to read, we can move onto the second step: looking at data globally to improve our accuracy through increased confidence.
One of the benefits of reading with a machine is that it is tireless. We can scour every page and every word, identifying and extracting every piece of data that might possibly be useful to us. Then, we can look for other instances of the same piece of data to increase our confidence that our data value is correct.
For example, consider the interest rate that appears on the Mortgage Note. How that interest rate is defined will vary depending on the home loan product, but with a fixed rate we know the figure should be consistent throughout the Note. If that’s the case, we increase our confidence score.
Across the entire package of documents needed to support the loan the interest rate is going to be mentioned a bunch of times. We can look at all instances of the interest rate anywhere it’s mentioned on any document. As we extract that value, we version it so we we’re seeing the latest instance, then we can compare that to the value we pulled off the Note. Again, consistency in these data increases our confidence score.
This contextual approach to data accuracy is intrinsic to TRUE technology. When we have multiple instances of the same piece of data, all cross-compared with each other, we can build a super plurality of that data value. When we compute this globally, we create a context that includes the super plurality of a specific value and its latest version. This reinforces our confidence in the accuracy of what we originally read off the Note because the entire loan package supports both the data value and the timeline.
An AI system can face similar confusion, but it has some advantages and disadvantages compared with the human reader. The processing power and perfect memory of a computer enables the AI to learn huge libraries of fonts. The machine can discern the different shapes of characters down to the level of a single pixel.
The corollary is that an AI can struggle when it encounters an unfamiliar font or when the resolution is poor (such as grainy, blurred, pixelated or obscured characters). To solve for this, we can use context (as with “Obviously” above). Does the character appear to be part of a word? What other instances of the character can we read from that page, or from the entire document? The more we learn about the environment of a page and across multiple pages, the more confident we can be about recognizing a single character.
The Art in Science
When data values are globally consistent, we can say we have a solid scientific base for accuracy.
Our data values have “provable correctness.”
So, what happens when this global analysis throws up an inconsistency? Suddenly, we have a problem with “the science” that supports our understanding of the world. We now need to make a judgement call on the errant piece of data; we’re back to the matter of gauging confidence.
Let’s consider another illustration. Think of an underwriter doing a stare-and-compare analysis of multiple documents to check that the borrower’s name matches. She might read my name several times and see the following:
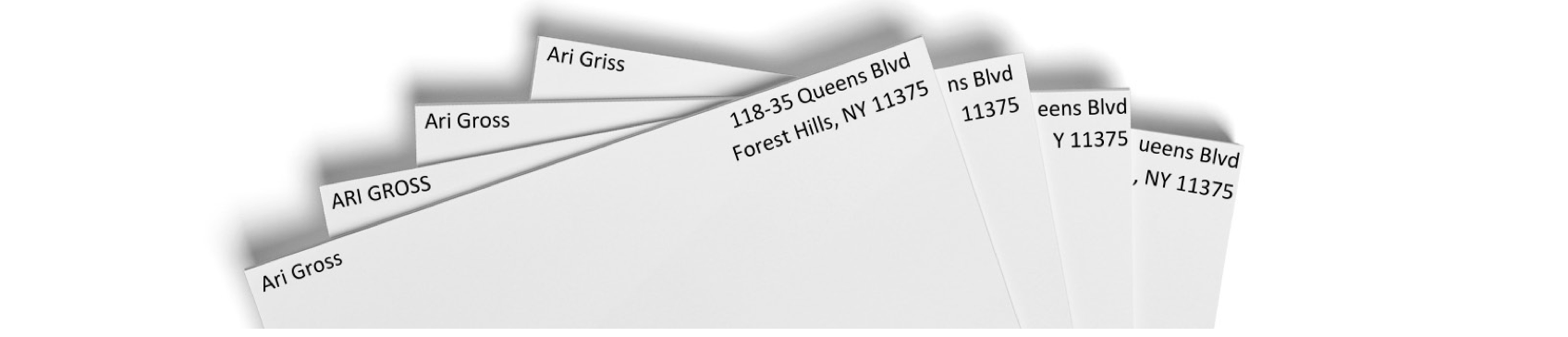
She understands that all-caps doesn’t change the data value, but that variance in spelling in the last example could be an issue. Is “Ari Griss” a different person or was this a typo? Looking further, she can see the consistency of related information, in this case the address. She also knows that “i” is next to “o” on a keyboard and that makes a typo more likely than, say, “Ari Gress.”
How can an AI achieve a similar, almost intuitive ability to know when not to react to inconsistent data?
It’s actually pretty easy. We can teach the AI the layout of a standard keyboard and common typing errors. When it sees an error that’s a match, it can return to that super plurality of data from our global comparison and decide to ignore the errant data value in favor of all the other instances. We can solve this problem by programming the AI to run similar comparative checks of related data – that global comparison.
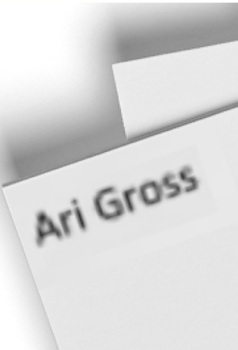
Let’s think of a tougher problem: poor print quality that makes “Ari Gross” hard to read.
Borrowers’ documents can quite often exhibit poor quality type. Documents can be damaged or marked before scanning. The scan itself may be suboptimal, with low resolution, blurring or bad alignment. As humans, we’re generally good at coping with this “noise.” How does our AI cope with this problem?
Here, TRUE has a unique advantage. I mentioned in the introduction that I began my AI research in the field of computer vision. I built technology that deconstructs how a document is made pixel by pixel. These techniques are the foundation of the
AI we’ve built at TRUE. Pixel-level analysis, plus training for virtually every font that exists, allows our OCR to cope with “noisy” reproduction of characters and still extract a data value. Our confidence in that value will score lower, but our contextual analysis of the page and document, plus our global comparison of all data, enables us to raise the confidence score.
Click through to view example documents
Provably Correct
No other AI system benefits from TRUE’s combination of sophisticated pixel-level OCR, training on thousands of fonts, pre-training on rich data sets of borrower documents, and both contextual and global data analysis. Training continues through each deployment, with the AI gaining in accuracy as it ingests the client’s borrower documents and system users make adjustments to suit their processes and preferences.
This layering of multiple AI technologies with human expertise and experience is how we achieve “provably correct” - lending intelligence where data and documents are directly and globally related and with confidence scores that satisfy requirements.
The result is an AI that significantly outperforms any human and delivers fully automated lending intelligence. Out-of-the-box we typically see document classification and data extraction accuracy rates starting around 94 percent, compared to around 80 percent with trained human agents.12 TRUE also outperforms AI- powered OCR systems from major technology brands, including Google and Amazon, as shown below.
Our combined passthrough rate – which measures data accuracy across an entire loan package not just single documents – is close to 90 percent. This is well above the requirements set by lenders and TRUE’s accuracy keeps on rising as our analytics engines are trained with a mortgage lender’s proprietary processes and customer data.
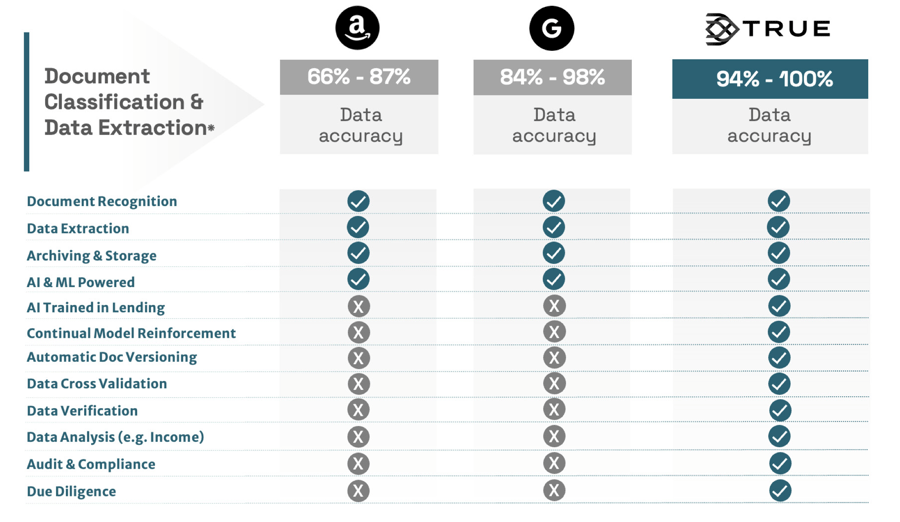
Hands Off
I’ve explained why accuracy is important, but there is an additional factor to consider when selecting an AI-enabled solution: is the data fully handled by AI or are there humans in the loop?
Many AI systems use human labor in the training stage, TRUE included, but some solution providers continue to use human labor as a standard part of their deployments. In instances where their AI struggles, human teams are on hand to improve data accuracy. This is not intrinsically bad, but it limits overall effectiveness.
-
Human intervention takes time. It slows down document classification, data extraction and verification processes, which limits key benefits of productivity and reduced time-to-close.
- Passing documents to external human teams is a data security risk. Mitigations can be put in place, but security professionals generally prefer sensitive data to stay within their own network.
TRUE has no humans in the loop; our system is 100 percent AI. That means processes are fully automated, with results delivered in minutes, and there is no external security risk.
I recommend putting solution providers to the test on both the accuracy and the integrity of their AI. We’ve developed an online test that allows you to see both the accuracy and fully-automated speed of TRUE for yourself. Click here to Try Now >>
Business Outcomes & Benefits
Once TRUE is deployed in a mortgage business, what outcomes and benefits should you expect? Our clients have different goals and challenges, but the following examples represent the projects and programs that have been their priorities.
Create an Elastic Business Model
An end to the perpetual challenge of matching staffing with volumes is a dream for operations leaders, but lending intelligence can finally make this happen. Volumes will continue to peak and trough, but automation of labor-intensive processes prevents lenders from having to add and subtract headcount or outsource work to BPO providers in response to shifting market conditions.
TRUE enables lenders to build truly elastic business models from the moment of deployment. Fees are set per loan, so costs match capacity as lenders scale up or down. In addition to costs, profit margins and service standards become more consistent and predictable.
Streamline & Standardize the Application Process
The application process is the beginning of a series of interactions between borrower and lender that adds up to the “customer experience.” Alignment between sales claims and reality will be tested; brand ratings will rise or fall.
“If you work with processors and underwriters, you’ll see that how they think and approach what they do is just so drastically different from one person to the next, and it’s a big problem for lenders,” said STRATMOR’s Fortier. “Adopting some consistency is really, really critical.”
AI-enabled automation of document classification and data extraction tasks ensures that initial underwriting is handled consistently, improving speed and quality at this stage of origination. TRUE solutions can be paired with POS systems to check that a complete and correct set of documents hasbeen provided. Gaps or errors are spotted in minutes, helping lenders to direct digital or human support at this crucial first stage.
Unlock Productivity
TRUE solutions dramatically increase productivity across data classification, extraction and verification tasks, but lending intelligence unlocks new productivity possibilities. As the AI processes documents, a clean and trusted data resource emerges. This allows managers to more fully exploit the productivity potential of existing investments, including LOSs and robotic process automation (RPA).
Lending intelligence can also improve the quality of work and employee satisfaction. “Would you rather be processing individual files or reviewing reports of hundreds of processed files?” asked Rich Swerbinsky, formerly the President and Chief Operating Officer at The Mortgage Collaborative, adding, “Anybody would prefer the latter.”
In addition to doing higher-value, less tedious work, lending professionals can use the time they win back to add value to the business. That includes optimization of business processes, increasing sales, and providing human support to customers when that is required.
Accelerate Product Innovation
“It’s not just the quality of the data, it’s how much is even collected,” said Kevin Peranio, Chief Lending Officer at PRMG, a residential mortgage lender.
Unlike manual data extraction, there is no limit to what data can be extracted from documents through AI-enabled automation. That allows lenders to gain proprietary data insights that give a more holistic view of the customer, enabling optimization of existing products, and prompting innovation.
Consider trends in work. We may have a general sense that more people are active in the gig economy, have more than one job, or independent side hustles. Lending intelligence will reveal insights from the data describing these trends, enabling product teams to better serve a changing market.
For example, a decade ago lenders might have applied more scrutiny to 1099 contractors because their income wasn’t as predictable as their W-2-only counterparts. Lending intelligence could show hidden strengths in earning patterns leading to more contractors being approved — a major change from years past. Understanding this, product teams might decide to take a data-driven approach and create mortgage products designed for borrowers who work 1099 jobs. With TRUE, extracting all data from every application, this kind of innovation becomes much easier and faster.
Strengthen the Customer Experience
According to a McKinsey report13, 42 to 67 percent of borrowers said they were not satisfied with their mortgage process (with banks performing worse compared to non-bank lenders).
Starting well, with streamlined and standardized applications, is important. However, there will always be exceptions and edge cases. Some customers may have problems with their documents, or they
may need specialist advice. The challenge for lenders is the time taken to handle exceptions, so a wise strategy is to use time savings from automation to redeploy staff.
“Getting that time back allows for exception processing on files that need a lot of manual hand-holding no matter what,” said Peranio. “Now, everybody gets more time to help that borrower, who oftentimes is a credit-challenged or even credit-invisible borrower.”
Satisfaction means more than the lowest price, but rising interest rates put pressure on lenders to be as competitive on rates and fees as possible. “When loans get cheaper, the consumer gets better rates, and everybody wins,” he added.
Gain Confidence in the Secondary Market
Lending intelligence increases confidence in loan quality between originators and the servicers and financers in the secondary market. TRUE solutions reduce defect rates to the lowest possible level – just fractions of a percent compared with 10 to 15 percent error rates in the early stages of manual processes.
Mortgage buybacks or putbacks are a huge loss for any lender and can be existential for some providers. “At the end of the day, the secondary market is driving all of the hesitation about data. Independent mortgage bankers have had a tough year, most have lost money, so the impact of having to buy loans back is greater,” said Swerbinsky.
TRUE solutions give originators complete confidence in the completeness and accuracy of data in their loan portfolios, significantly reducing the risk of buybacks. Secondary market organizations can use the same solution to switch auditing of incoming portfolios from a sample to as much as 100 percent of loans without needing any additional time. Deals close quicker and loans are securitized faster, further reducing market risk.
“The thought that ‘wait a minute, my data doesn’t equal my docs’ kind of goes away. I’m already hearing from folks that they’re seeing several hundred dollars per loan alone improvement,” said Rick Hill, Vice President of Information Technology at the Mortgage Bankers Association.
Digital Detection of Fraud
The high value of homes means mortgage fraud can be attractive to individuals, organized crime, and corrupt mortgage industry workers acting in collusion. Research shows that 1 in every 131 mortgage applications shows signs of fraud.
Global data comparison is a powerful tool for fraud detection. It takes an exceptionally skilled and diligent fraudster to ensure doctored data is consistent across a large and complex data file and its underlying documents. Nevertheless, we should not assume complexity itself is sufficient deterrent.
TRUE applies pixel-level analysis, an intrinsic ability of our AI methodology, to provide a deeper layer of fraud detection. Our knowledge of fonts and page construction allows TRUE to detect the slightest evidence of document manipulation, well beyond the ability of the human eye. Without revealing too much, our anti-fraud system will warn of variations in fonts, the positions of characters in relation to other characters, traces of characters that have been removed, the introduction of new characters, and irregular resolution that suggests interference.
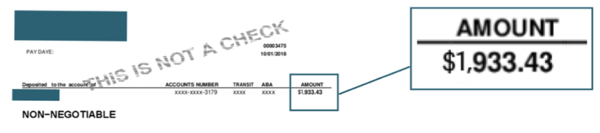
To the human eye, the dollar amount looks fine. However, at the pixel level font manipulation becomes clear.
Our AI Future
I used the term ‘disruption’ to connote the scale of change that I predict AI will bring to the mortgage industry. Virtually all industries are being altered by AI in some way, so why is such a term justified?
Remember the example of the credit card industry, where easy availability of structured and trusted data has led to a proliferation of competitors and consumer choice? A market that was once ruled by mainstream banks is now open to a vast array of non-banking brands. Credit-led strategies are even challenging the banks directly, with “neobanks” entering the market by offering a credit card and then developing bank account products in quick succession.
The mortgage industry’s data problem (difficulties and delay extracting structured data from unstructured documents) is one that is being solved by AI. From airlines to retail, we’ve witnessed improvements in technology lead to radically altered markets. There is no reason why AI could not catalyze similar change in mortgage lending and a produce a consequent proliferation of competitors and consumer choice.
"AI Won't Replace Humans...
...But humans with AI will replace humans without AI.” So ran a recent headline about the commercial and societal impact of AI in Harvard Business Review. It’s a fool’s game to make confident predictions about AI the future of work, but we know this is not the first time humanity has seen work disappear to automation.
The mechanical loom decimated home-weaving. The motor car almost eliminated jobs associated with horse-drawn transportation. The photocopier and personal computer put typing pools firmly in the past. And yet, whatever the innovation, the world after has invariably benefited from new jobs, an increase in work, higher productivity, and economic growth.
Could it be different this time? Is there something more destructive to jobs with the automation of intelligence compared to human labor? Again, the evidence suggests not. AI is already doing many “human” jobs, from diagnosing disease to customer service, but demand for medical and interpersonal skills is growing not declining. Macro factors increasing demand for workers include widespread labor shortages and aging populations in both advanced and emerging economies.
Mortgage Industry Jobs
If the broader jobs market is not presently a concern, is there a specific threat from AI to mortgage industry jobs? According to HousingWire, “a rapid rise in mortgage rates and a big drop in origination volume has led to thousands of industry job losses in 2022 and 2023.” “We’ve seen the extremes these last three years,” said Swerbinsky. “I would estimate 40 percent of the employees that were in the mortgage industry a year ago are now gone.”
The anxiety for lenders is therefore less about cutting jobs, but whether AI can enable trimmed down businesses to avoid a return to the cycle of job creation and destruction.
For TRUE clients, this future has already come to pass. They have adapted business processes, integrating AI and human skills to improve performance, elasticity and job satisfaction.
“I think decision makers are of the mindset that ‘I can’t continue to add and subtract human bodies like this anymore.
One, it’s inefficient. Two, there’s the human side of it, unless you’re a cold-hearted killer.”
TRUE AI and Mortgage Lending
This paper began with two simple questions. Why AI? Why Now?
The answer to the first is that AI that delivers lending intelligence can solve the mortgage industry’s data problem. But what about the second question?
If you are a leader in the mortgage industry, the potential of AI and its return on investment (ROI) is becoming an increasingly urgent matter. This is because AI holds huge disruptive potential: many stakeholders in the mortgage industry understand that this is technology that cannot be ignored.
I’d go so far as saying that AI-led disruption is set to define the future winners and losers in the mortgage industry. The challenge facing you as a leader is where to place your AI bets to ensure your business emerges as one of the winners.
The Industry View
At this point, let me highlight research carried out by TRUE that provides an independent industry perspective. We interviewed a panel of mortgage industry luminaries for a white paper about the need for and application of lending intelligence.
The Experts' View
One of the most vexed questions with any technology investment is ROI. Most assessments are limited to an analysis of cost savings, efficiency gains, or productivity improvements. These are important metrics, and lending intelligence will improve all of them, but total ROI is broader-based and accumulates over time.
TRUE commissioned experts in the fields of AI and total cost of ownership modelling to assess the bigger picture. All are former analysts with Gartner and Forrester Research. They presented their findings in a report: The Continuously Improving ROI of Trusted Data.
Put TRUE to the Test
I’m a big believer in testing. It’s how we train AI systems in the lab and prove their performance through product development and deployment. The challenge for those investing in AI is that it can be something of a “black box” – you can see the inputs and outputs but the internal workings are opaque.
In most instances, the only way to test an AI system prior to purchase and deployment is to run demos and technical evaluations. I’ve been a buyer of technology enough times to know that there’s always that nagging concern that I’m being presented with an idealized experience.
At TRUE, we’ve sought to increase transparency by opening our AI for direct external testing. Our
free, web-based tool allows you to upload your own set of borrowers’ documents and see how TRUE technology performs without any preparation or adjustments by us. In real time and with no humans in the loop, you’ll be presented with a report showing document classification and data extraction results – the basis of lending intelligence.
Further Reading
Market trends, industry reports, customer case studies and AI insights to help you navigate digital transformation, innovation and next-gen mortgage technologies.
Download full report
Click through to download our full AI Whitepaper in a PDF version.